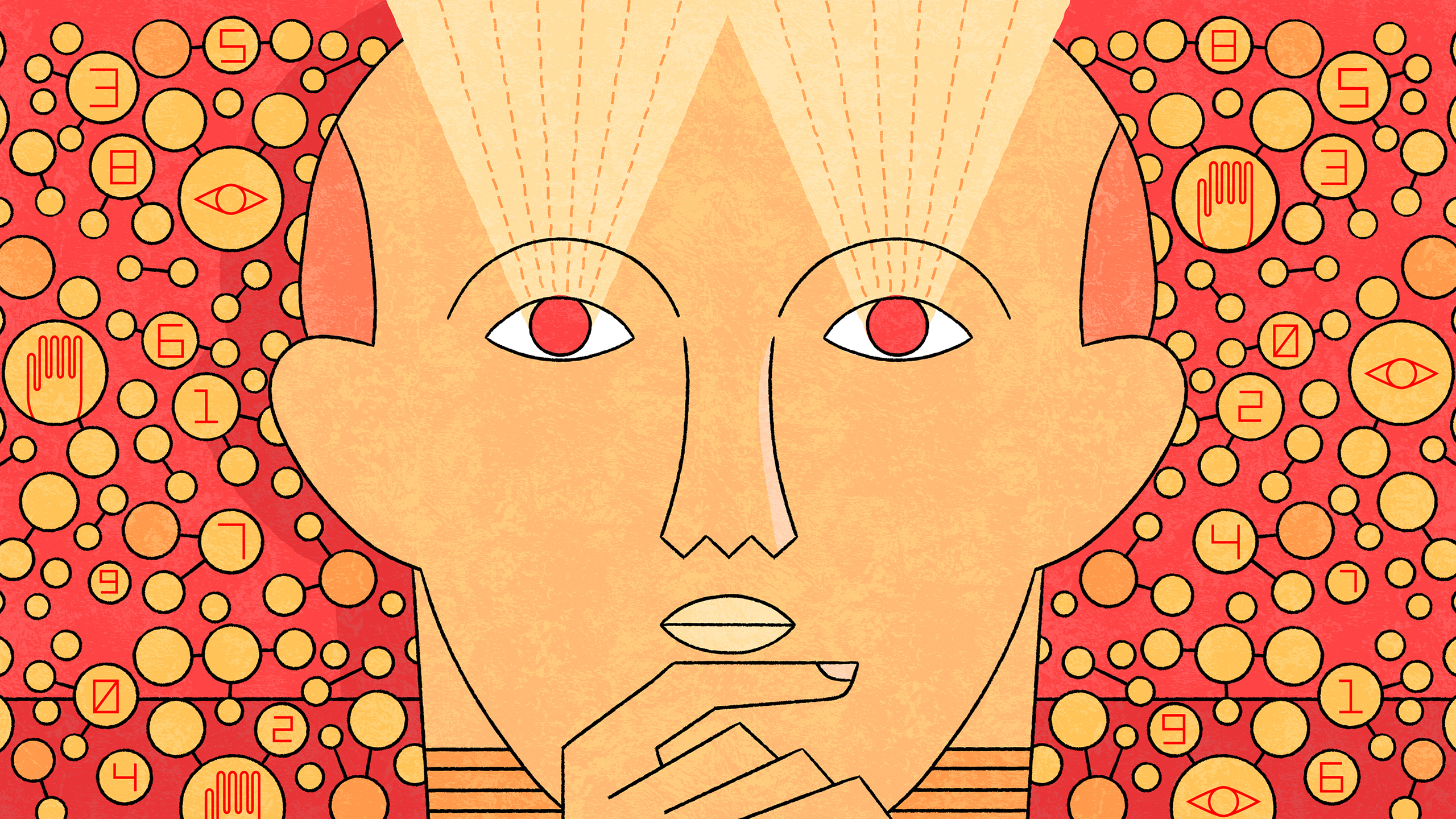
James O’Brien for Quanta Magazine
You don’t typically build a machine without understanding how it works. But for artificial intelligence researchers building large language models, understanding is about the one thing they haven’t achieved. In fact, sometimes their work feels more like gardening than engineering.
“Put a tomato seed into the ground and you get a tomato plant,” said Martin Wattenberg, a language model researcher at Harvard University. “You watered it, you weeded around it, but how on earth does that tomato plant work?”
Some scientists study language models by observing how they respond to different prompts — an approach akin to behavioral psychology. Researchers in the burgeoning subfield of mechanistic interpretability, inspired by neuroscience, instead try to understand models by opening them up and poking around inside. Their early efforts have already helped explain how language models represent concepts and how they accomplish certain simple tasks. They’ve also revealed some surprises that demonstrate how tricky it can be to truly understand AI.
Large language models are built around mathematical objects loosely based on the structure of the human brain. Known as artificial neural networks, they chain together many simple mathematical operations, processing strings of numbers that represent words. Whether a language model responds to prompts with gibberish or uncanny fluency depends on another set of numbers called parameters, which describe the connections inside its neural network. Large language models can have billions or even trillions of parameters, and researchers have no idea how to choose a good set of values in advance. Instead, they start with random ones, then give the model a ton of data and a simple objective: Given any snippet of text from this data set, predict the next word.
The model repeats this word prediction task trillions of times. After each attempt, a separate algorithm nudges the model’s parameters in a direction that makes the correct answer slightly more likely. This process is called training, but that’s something of a misnomer. Once researchers set it in motion, they’re about as involved in the model’s development as a gardener watching a tomato plant grow.
In theory, researchers can peer inside a fully trained language model and read out the values of all its parameters. They can also measure how a model responds to any specific prompt by recording the output, or “activation,” of each of its internal components. Together, these provide a wealth of data that any neuroscientist would envy — analogous to a perfect map of a person’s brain, along with separate electrodes to monitor the activity of each neuron. But all these numbers don’t add up to an explanation. Good luck using them to predict how the model will respond to new prompts.
Fortunately, interpretability researchers can do more than just read the values of parameters and activations: They can also alter them. Editing parameters is akin to ultra-targeted brain surgery — a scalpel capable of tweaking single neurons. Editing activations lets researchers temporarily change a specific component’s response to any given stimulus, to see how that affects the model’s output.
Activation editing also allows researchers to do something akin to copying and pasting mental states: They give a model one prompt, record the activations of certain components, then insert those activations into the model’s response to a second prompt. Researchers have used this technique to pinpoint where certain facts are stored in a language model. But such results aren’t always straightforward. Even with strong evidence that a concept is stored in one part of a model, it’s sometimes possible to alter its knowledge of that concept by tinkering in another part. It’s one of many cases where the inner workings of neural networks defy human intuition.
“There are so many things that seem like they should definitely be true, but when you take a closer look, they just aren’t,” said Asma Ghandeharioun, an interpretability researcher at Google DeepMind.
Researchers have also made progress identifying the procedures that language models use to perform tasks such as retrieving relevant words from earlier in a sentence, identifying the grammatical function of certain words or doing simple arithmetic. They’ve observed that sometimes models follow different procedures for variations of the same task, in ways that feel arbitrary. It’s like checking the weather before you brush your teeth, because if it’s raining you always use a hot pink toothbrush. In other cases, researchers have found that models contain many independent clusters of components doing exactly the same thing, which can confound efforts to tease apart the effects of different components. They’ve even observed an “emergent self-repair” phenomenon, where deactivating part of a model caused other components to change their behavior and take on the functions of the part that was turned off.
Despite these challenges, many interpretability researchers remain cautiously optimistic about the field’s prospects. “It is possible to make progress,” Wattenberg said. “We’re well ahead of where we were five years ago.”