For Persi Diaconis’ Next Magic Trick …
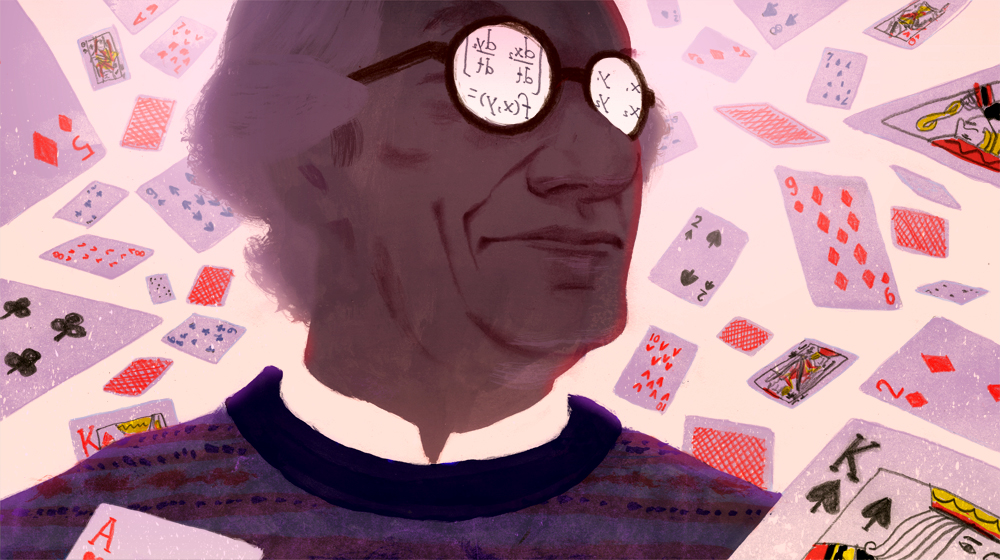
Persi Diaconis shuffled and cut the deck of cards I’d brought for him, while I promised not to reveal his secrets. “I’m not going to give you the chance,” he retorted. In an empty conference room at the Joint Mathematics Meetings in San Antonio, Texas, this January, he casually tossed the cards into four piles in a seemingly random motion — yet when he checked, each pile magically had an ace on top. “Of course, it’s easy to get confused when there are a lot of cards, so let me just take four,” he said, scooping up the aces. He swiveled the four-card pile in his hands — always keeping it in the same flat plane — and sometimes the aces were faceup, sometimes facedown, even though they couldn’t possibly have flipped over.
Diaconis’ career as a professional magician began more than five decades ago, when he ran away from home at age 14 to go on the road with the sleight-of-hand virtuoso Dai Vernon. But unlike most magicians, Diaconis eventually found his way into academia, lured by an even more powerful siren song: mathematics. At 24, he started taking college classes to try to learn how to calculate the probabilities behind various gambling games. A few years later he was admitted to Harvard University’s graduate statistics program on the strength of a recommendation letter from the famed mathematics writer Martin Gardner that said, more or less, “This kid invented two of the best ten card tricks in the last decade, so you should give him a chance.”
Now a professor of mathematics and statistics at Stanford University, Diaconis has employed his intuition about cards, which he calls “the poetry of magic,” in a wide range of settings. Once, for example, he helped decode messages passed between inmates at a California state prison by using small random “shuffles” to gradually improve a decryption key. He has also analyzed Bose-Einstein condensation — in which a collection of ultra-cold atoms coalesces into a single “superatom” — by envisioning the atoms as rows of cards moving around. This makes them “friendly,” said Diaconis, whose speech still carries the inflections of his native New York City. “We all have our own basic images that we translate things into, and for me cards were where I started.”
In 1992, Diaconis famously proved — along with the mathematician Dave Bayer of Columbia University — that it takes about seven ordinary riffle shuffles to randomize a deck. Over the years, Diaconis and his students and colleagues have successfully analyzed the effectiveness of almost every type of shuffle people use in ordinary life.
All except one: “smooshing.”
This toddler-level technique involves spreading the cards out on a table, swishing them around with your hands, and then gathering them up. Smooshing is used in poker tournaments and in baccarat games in Monte Carlo, but no one actually knows how long you need to smoosh a deck to randomize it. “Smooshing is a completely different mechanism from the other shuffles, and my usual techniques don’t fit into that,” Diaconis said. The problem has tantalized him for decades.
Now he is on a quest to solve it. He has carried out preliminary experiments suggesting that one minute of ordinary smooshing may be enough for all practical purposes, and he is now analyzing a mathematical model of smooshing in an attempt to prove that assertion.
Diaconis’ previous card-shuffling work has helped to shed light on numerical approximation algorithms known as Markov chain Monte Carlo methods, ubiquitous in scientific simulation, which employ processes akin to shuffling to generate random examples of phenomena that are too hard to calculate completely. Diaconis believes that a mathematical analysis of smooshing will likewise have ramifications that go far beyond card shuffling. “Smooshing is close to a whole raft of practical life problems,” he said. It has more in common with a swirling fluid than with, say, a riffle shuffle; it’s reminiscent, for example, of the mechanics underlying the motion of large garbage patches in the ocean, during which swirling currents stir a large collection of objects.
“The smooshing problem is a way of boiling down the details of mixing to their essence,” said Jean-Luc Thiffeault, a professor of applied mathematics at the University of Wisconsin, Madison, who studies fluid mixing.
Fluid-flow problems are notoriously hard to solve. The most famous such problem, which concerns the Navier-Stokes equations of fluid flow, is so difficult that it has a million-dollar bounty on its head. “The mathematics of any model for spatial mixing is in pretty bad shape,” Diaconis said.
Diaconis hopes that the union of fluid-flow techniques and card-shuffling math might point a way forward. “My kind of math — combinatorics, probability — is at right angles to the kind of math the Navier-Stokes people do,” he said. “If you bring fresh tools in, it might do some good in a bunch of these classical problems.”
Going Random
It might seem that no amount of smooshing can be definitively determined to be enough. After all, no matter how long you’ve smooshed the cards, wouldn’t more smooshing be even better? From a practical standpoint, probably not. Diaconis and Thiffeault both suspect that there is a particular moment in smooshing — a “cutoff,” as mathematicians call it — at which the deck transitions from highly ordered to highly unpredictable. After this point, more smooshing will confer only inconsequentially tiny increments of additional randomness.
The cutoff phenomenon, which occurs in a variety of situations in math and physics, owes its discovery to an earlier shuffling analysis by Diaconis and Mehrdad Shahshahani. In 1981 the pair was trying to understand a simple shuffle in which you just swap two randomly chosen cards. If you do many such shuffles, for a long time the deck will be far from random. But after roughly 100 shuffles it will suddenly transition to nearly random.
Since that discovery, the cutoff has been identified in many Markov Chain Monte Carlo algorithms, and recently it has even been discovered in the behavior of atomic spins in the Ising model, which describes the process by which materials become permanent magnets. “The idea of the cutoff has been very influential,” said Yuval Peres, a mathematician at Microsoft Research in Redmond, Wash.
All the card shuffling methods that have been successfully analyzed have cutoffs, and Diaconis conjectures that smooshing will too. “I’d bet $100 to $1 that smooshing has a cutoff,” Diaconis said.
10 Smooshing Tests
Diaconis is drawn to problems he can get his hands on. When he got curious about how shaving the side of a die would affect its odds, he didn’t hesitate to toss shaved dice 10,000 times (with help from his students). And when he wondered whether coin tossing is really unbiased, he filmed coin tosses using a special digital camera that could shoot 1,000 frames per second — and discovered, disconcertingly, that coin tosses are slightly biased toward the side of the coin that started out faceup.
So to get a feel for how much smooshing is needed to produce a random deck, Diaconis grabbed a deck and started smooshing. Together with his collaborators, the Stanford biostatistician Marc Coram and Lauren Bandklayder, now a graduate student at Northwestern University, he carried out 100 smooshes each in lengths of 15 seconds, 30 seconds, and one minute.
Next, he had to figure out how random the decks had become. The ideal way to do this would be to check whether each possible deck arrangement appears equally often among the smooshed decks. But this approach is utterly impractical: The number of arrangements of a deck of cards is 52 factorial — the product of the first 52 numbers — which approaches the estimated number of atoms in the Milky Way galaxy. “If everyone had been shuffling decks of cards every second since the start of the Earth, you couldn’t touch 52 factorial,” said Ron Graham, a mathematician at the University of California, San Diego. In fact, any time you shuffle a deck to the point of randomness, you have probably created an arrangement that has never existed before.
Since a direct experimental test of randomness isn’t feasible, Diaconis and his collaborators subjected their smooshed decks to a battery of 10 statistical tests designed to detect nonrandomness. One test looked at whether the top card of the deck had moved to every possible position equally often in the 100 smooshed decks. Another looked at how often pairs of adjacent cards — the seven and eight of spades, for example — remained adjacent after the shuffle.
Of the 10 tests, Diaconis suspected that smooshing might have the hardest time passing the adjacent-pairs test, since cards that start out together might get swept along together by the hand motions. And indeed, the 15-second smooshes failed the adjacent-pairs test spectacularly, often having as many as 10 pairs still adjacent after the smoosh — more than enough hidden order for a smart gambler to exploit. “If you know that, say, 10 percent of the cards are still going to be next to the cards they were next to before, that’s a tremendous advantage if you’re playing blackjack,” Graham said.
Diaconis expected the 30-second and one-minute smooshes to fail the adjacent-pairs test too, but to his surprise, they aced all 10 tests. “I thought this was a lousy method of shuffling,” he said. “I have new respect for it.”
The experiments don’t prove that 30 seconds is enough smooshing to randomize a deck. They only establish that 30-second smooshes are not as egregiously nonrandom as 15-second smooshes. With a sample size of only 100 smooshes, “you can only detect very strong departures from randomness,” Diaconis said. It seems likely that the cutoff occurs sometime before one minute, since 30-second smooshes already seem to do pretty well. But, he said, “we’d be on more solid ground in discriminating between 30 seconds and one minute if we had 10,000 smooshes.” That’s far more than his group can carry out, so Diaconis is thinking about organizing a “national smoosh” in high-school or junior-high math classes.
Even more than additional data, however, Diaconis wants a proof. After all, ad hoc statistical tests are never a conclusive way to show that a shuffle is random. “It’s perfectly possible that some clever person will say, ‘Why didn’t you try this test?’ and it turns out to all be wrong,” he said. “I want to be able to say, ‘It doesn’t work after a minute and here’s why,’ or ‘It works after a minute and here’s a proof.’”
Theoretical Smooshing
When Diaconis returned to college after a decade as a professional magician, his first three grades in advanced calculus were C, C and D. “I didn’t know you were supposed to study,” he said. His teacher told him that he should write down the proofs and practice them as if they were French verbs. “I said, ‘Oh, you’re allowed to do that?’” Diaconis said. “I thought you were just supposed to see it.”
When it came to smooshing, instead of just trying to “see it,” Diaconis devoured the literature on fluid mixing. “When we started talking about the connections between cards and fluid mixing, he read the whole 200 pages of my Ph.D. thesis,” said Emmanuelle Gouillart, a researcher who studies glass melting at Saint-Gobain, a glass and construction materials company founded in Paris in 1665. “I was really impressed.”
While Diaconis grew more conversant in fluid mechanics, Gouillart benefited from his unique insight into card shuffling. “It turned out that we were studying very similar systems, but with different descriptions and different tools,” Gouillart said. The collaboration led her to develop a better way to measure correlations between neighboring particles in the fluids she studies.
Diaconis, meanwhile, has developed a mathematical model for what he calls “the sound of one hand smooshing.” In his model, the cards are represented by points scattered in a square, and the “hand” is a small disk that moves around the square while rotating the points under it by random angles. (It would be easy, Diaconis noted, to extend this to a two-handed smooshing model, simply by adding a second disk.)
Diaconis has been able to show — not just for a 52-card deck but for any number of points — that if you run this smooshing model forever, the arrangement of points will eventually become random. This might seem obvious, but some shuffling methods fail to randomize a deck no matter how long you shuffle, and Diaconis worried that smooshing might be one of them. After all, he reasoned, some cards might get stuck at the edges of the table, in much the same way that, when you mix cake batter, a little flour inevitably gets stranded at the edges of the bowl and never mixes in. But by drawing on 50 years of mathematics on the behavior of random flows, Diaconis proved that if you smoosh long enough, even cards at the edge will get mixed in.
His theoretical result says that the smooshing model will eventually mix the cards, but doesn’t say how long it will take. The model does provide a framework for relating the size of the deck to the amount of mixing time needed, but pinning down this relationship precisely requires ideas from a mathematical field still in its infancy, called the quantitative theory of differential equations. “Most studies of differential equations focus on what happens if you run the equation for a long time,” Diaconis said. “People are just now starting to study how the equation behaves if you run it for, say, a tenth of a second. So I have some careful work to do.”
Diaconis is optimistic that the work will lead him not just to an answer to the smooshing question, but to deeper discoveries. “The other shuffles have led to very rich mathematical consequences, and maybe this one will too,” he said.
Diaconis shares his magical secrets with only a select inner circle, but he dreams of laying the secrets of smooshing bare. “Smooshing is something that people use thousands of times a day, and mathematicians should be able to say something about it.”