The Hard Lessons of Modeling the Coronavirus Pandemic
Introduction
For a few months last year, Nigel Goldenfeld and Sergei Maslov, a pair of physicists at the University of Illinois, Urbana-Champaign, were unlikely celebrities in their state’s COVID-19 pandemic response — that is, until everything went wrong.
Their typical areas of research include building models for condensed matter physics, viral evolution and population dynamics, not epidemiology or public health. But like many scientists from diverse fields, they joined the COVID-19 modeling effort in March, when the response in the United States was a whirlwind of “all hands on deck” activity in the absence of any real national direction or established testing protocols. From the country’s leaders down to local officials, everyone was clamoring for models that could help them cope with the erupting pandemic — hoping for something that could tell them precisely how bad it would get, and how quickly, and what they should do to head off disaster.
In those early months of 2020, Goldenfeld and Maslov were showered with positive press coverage for their COVID-19 modeling work. Their models helped prompt their university to shut its campus down quickly in the spring and transition to online-only education. Shortly thereafter, theirs was one of several modeling groups recruited to report their results to the office of the governor of Illinois.
So when Goldenfeld, Maslov and the rest of their research team built a new model to guide their university’s reopening process, confidence in it ran high. They had accounted for various ways students might interact — studying, eating, relaxing, partying and so on — in assorted locations. They had estimated how well on-campus testing and isolation services might work. They had considered what percentage of the student body might never show symptoms while spreading the virus. For all those factors and more, they had cushioned against wide ranges of potential uncertainties and hypothetical scenarios. They had even built in an additional layer of detail that most school reopening models did not have, by representing the physics of aerosol spread: how many virus particles a student might emit when talking through a mask in a classroom, or when drinking and yelling above the music at a crowded bar.
Following the model’s guidance, the University of Illinois formulated a plan. It would test all its students for the coronavirus twice a week, require the use of masks, and implement other logistical considerations and controls, including an effective contact-tracing system and an exposure-notification phone app. The math suggested that this combination of policies would be sufficient to allow in-person instruction to resume without touching off exponential spread of the virus.
But on September 3, just one week into its fall semester, the university faced a bleak reality. Nearly 800 of its students had tested positive for the coronavirus — more than the model had projected by Thanksgiving. Administrators had to issue an immediate campus-wide suspension of nonessential activities.
What had gone wrong? The scientists had seemingly included so much room for error, so many contingencies for how students might behave. “What we didn’t anticipate was that they would break the law,” Goldenfeld said — that some students, even after testing positive and being told to quarantine, would attend parties anyway. This turned out to be critical: Given how COVID-19 spreads, even if only a few students went against the rules, the infection rate could explode.
Critics were quick to attack Goldenfeld and Maslov, accusing them of arrogance and failing to stay in their lane — “a consensus [that] was very hard to beat, even when the people who are recognized experts chimed in in our defense,” Maslov said.
Meanwhile, the University of Illinois was far from unique. Many colleges across the country were forced to reckon with similar divergences between what their own models said might happen and what actually did happen — divergences later attributed to a wide-ranging assortment of reasons.
Such events have underscored a harsh reality: As incredibly useful and important as epidemiological models are, they’re an imperfect tool, sensitive to the data they employ and the assumptions they’re built on. And because of that sensitivity, their intended aims and uses often get misunderstood.
The researchers developing these models must navigate unfathomably difficult terrain, providing answers where often there are none, with a certainty they can’t guarantee. They must make assumptions not only about the virus’s biology, which remains far from fully understood, but about human behavior, which can be even more slippery and elusive. And they have to do it all at warp speed, cramming weeks’ or months’ worth of analysis into a matter of days without sacrificing accuracy. All the while, they are suffering the long hours, sleepless nights and personal toll of acting as scientist, communicator, advocate and adviser all in one. (Not to mention their usual responsibilities, also magnified manyfold by the crisis: On calls with many of these scientists, there’s a good chance of hearing infants crying, dogs barking or a cacophony of other activity in the background.)
“Right now, I’m just really tired,” said Daniel Larremore, a computational biologist at the University of Colorado, Boulder, whose modeling efforts included plotting out scenarios for his college’s campus reopening. “There’s no clear best solution, and people are going to get sick. And I just wonder how it [will feel] when one of my colleagues gets really sick, or if somebody dies.” He remembers that after he and others in the same position confessed to that exhaustion on Twitter, someone retorted that these stakes are nothing new to people working in national security and defense fields — but that doesn’t help with the strain of facing grim choices and having to look for the least intolerable among them.
“You do the very best you can with what you’re handed, but I don’t know,” he said. “I look at the numbers that come in every day and wonder … what are we missing.”
In the global health crisis wrought by COVID-19, epidemiological modeling has played an unprecedented role — for scientists, decision-makers and the public alike. Just over a year ago, terms like “reproduction number” and “serial interval” were anything but common phrases for the average person. Even in the scientific community, infectious disease modeling has been “a pretty niche field of research,” said Samuel Scarpino, a network scientist and epidemiologist at Northeastern University.
Over the past year, models have opened a window into the inner workings of the novel coronavirus and how it spreads. They have offered well-focused snapshots showing the impact of the disease at given instants in myriad settings, and suggested how those situations might change going forward. They have guided decisions and policies, both for shutting down parts of society and for opening them back up.
At the same time, scientists have had to reckon with the limitations of models as tools — and with the realization that pandemics can push the utility of models to the breaking point. The ravages of disease on society intensify the headaches involved in obtaining unbiased, consistent patient data, and they amplify the fickleness and irrationality of human behaviors that the models need to mirror. Perhaps the greatest challenge of all is simply making sure that decision-makers fully understand what the models are and are not saying, and how uncertain their answers are.
But those challenges are also driving huge improvements. The world of epidemiological modeling has seen “a lot of new thinking, new methods,” said Lauren Ancel Meyers, a mathematical biologist at the University of Texas, Austin. “I would venture that we’ve probably progressed as much in the last [10] months as we have in the prior six years.”
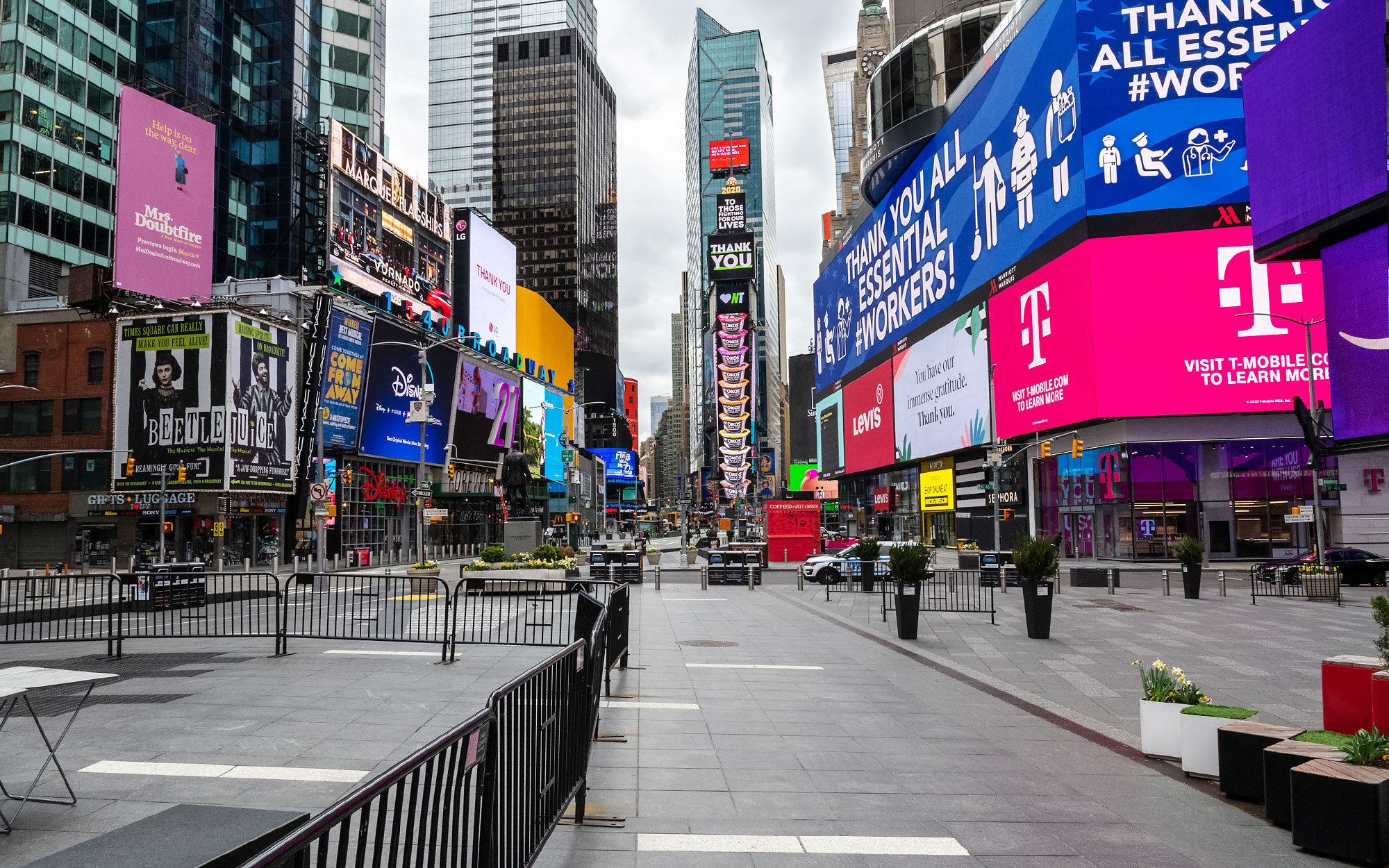
Times Square during the pandemic in New York City. Many people fled urban centers or isolated themselves even before governments started ordering that schools and businesses shut down. Researchers hope to analyze data to disentangle the effects of these mitigation measures to guide future policy.
Diagnosing a Pandemic
When infectious disease specialists began hearing in late December 2019 and early January 2020 that a cluster of pneumonia-like illnesses had cropped up in China, they instantly became watchful. “In our field,” said Adam Kucharski, an epidemiologist at the London School of Hygiene and Tropical Medicine, “a pandemic is always on the radar as a threat.” Indeed, four epidemics — H1N1 influenza, SARS, MERS and Ebola — flared up during the past couple of decades alone, and new infectious agents are constantly evolving.
But in those earliest days of the coronavirus outbreak in China, no one knew enough about it to anticipate whether it would erupt into a threat or quickly fade away, as so many diseases do. Was the virus being transmitted person to person, or had all the infections arisen from the same animal sources at one marketplace in Wuhan? Could shutting down borders contain the infection, or might it spread globally?
With virtually no data in hand, and no real concept yet of what the virus was doing or how it worked, researchers looked to models for answers.
The mathematical disease modeler Joseph Wu, a member of the University of Hong Kong’s infectious disease and public health teams, was looking closely at the numbers of cases being reported in and around Wuhan. Human-to-human transmission had started to seem extremely likely: A handful of cases had cropped up beyond China’s borders, first in Thailand, then in Japan. Based on the reported spread and the travel volume from China to other countries, Wu said, he and his colleagues figured that “the number of infected cases in Wuhan must be much, much larger than what they had announced at that time.” A significantly larger outbreak size would mean that people were passing the virus on to others.
Wu and his team weren’t the only ones reaching those conclusions. On January 10, 2020, the World Health Organization hosted a teleconference for teams of experts around the globe who were doing similar analyses, pulling together their own models of the disease and voicing the same concerns.
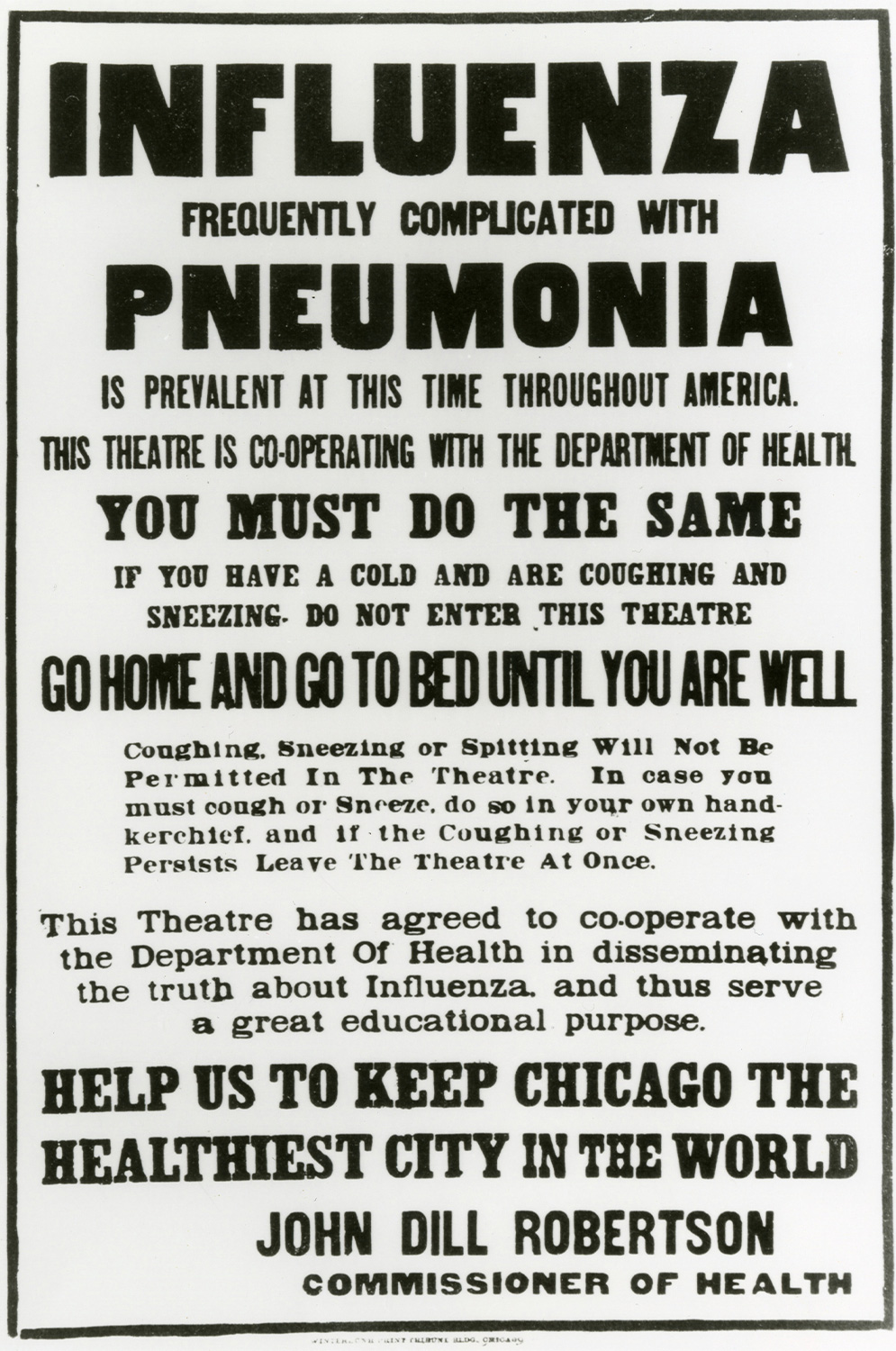
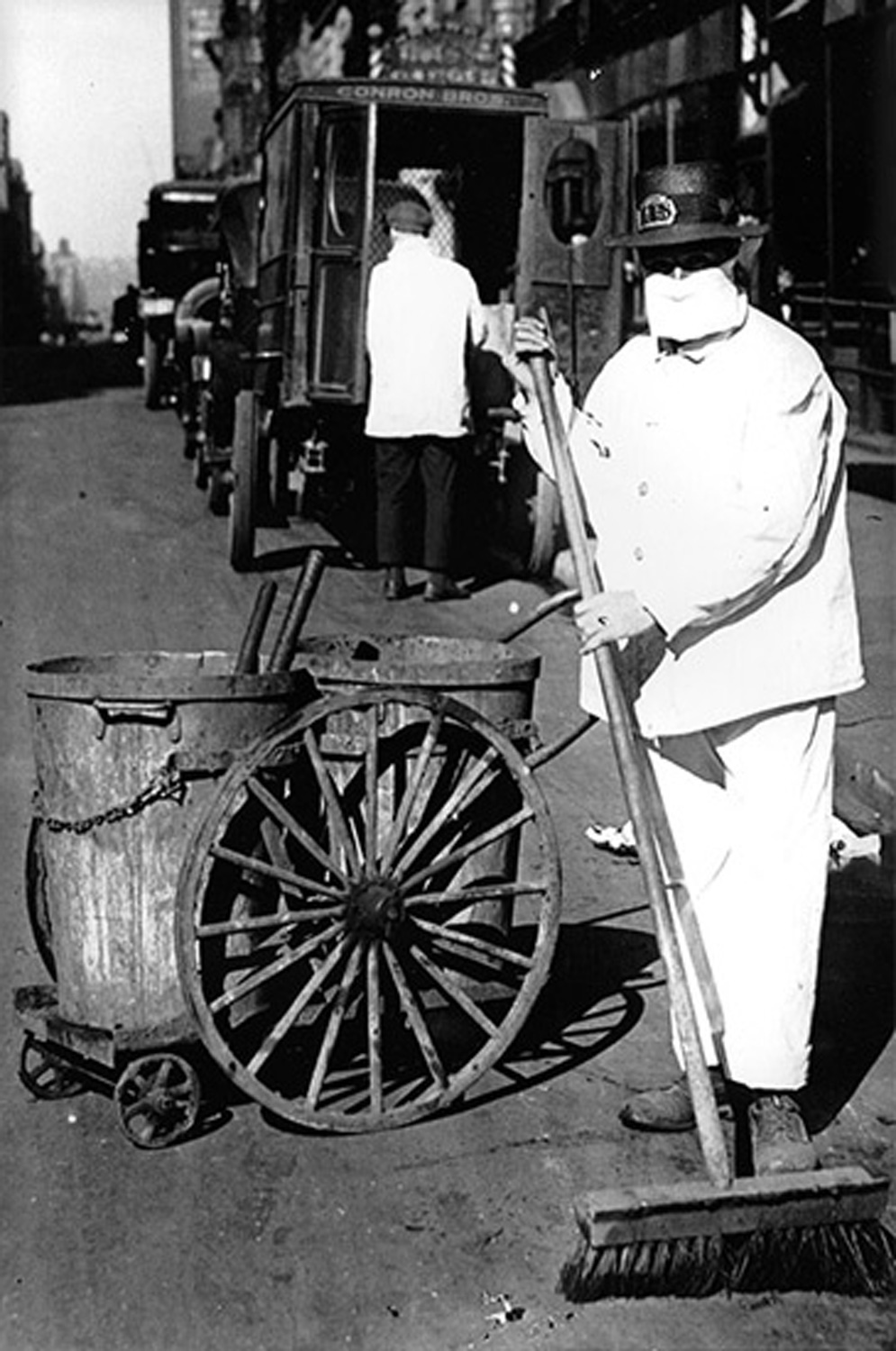
The COVID-19 pandemic has been compared to the 1918 influenza pandemic, despite the dissimilarities in the viruses. Then, as now, social distancing was a major part of the public response (top), and civilians and essential workers like this New York City street sweeper had to wear masks.
The COVID-19 pandemic has been compared to the 1918 influenza pandemic, despite the dissimilarities in the viruses. Then, as now, social distancing was a major part of the public response (as shown in the poster at left), and civilians and essential workers like this New York City street sweeper (right) had to wear masks.
Still, the Hong Kong group wanted more data to get a better grasp of the situation. On January 23, 2020, Wu and his colleagues boarded a flight to Beijing, with plans to meet with members of the Chinese Center for Disease Control and Prevention.
They made it just as the crisis came to a head: While they were in the air, Wuhan went into official lockdown. (They learned the news only after their plane landed.) After several hectic days collaborating with researchers at the Chinese CDC who had spent the previous three weeks gathering data, the Hong Kong delegation concluded that the outbreak in Wuhan must have been on the order of tens of thousands of cases, not the couple thousand that had been officially reported at that point. “Given that they were locking down the city even before we saw the data,” Wu said, “we sort of already knew.”
Even more concerning, those numbers and subsequent analyses implied not only that the virus could spread rapidly but that a large percentage of cases were going undetected, slipping through the cracks and seeding epidemics elsewhere. Much of the transmission was happening even before people started to show symptoms. It was time to make the call that this was a pandemic — one that was not only swift, but also at least partly silent.
“Imagine rewinding back to the end of January, when it was mostly just Wuhan,” Wu said. “When we make that statement, there’s some pressure: What if we were wrong?” They could be setting off a global panic needlessly. And maybe worse, they could be damaging how epidemiological models might be received in the future, setting up more public health crises in years to come. In the end, they decided that the risk was great enough “that we should go forward and warn the world, even though we know that there’s some uncertainty in our predictions,” he said.
“As a researcher, of course I hope to contribute,” he added. “But at the same time, I’m not used to taking that kind of responsibility.”
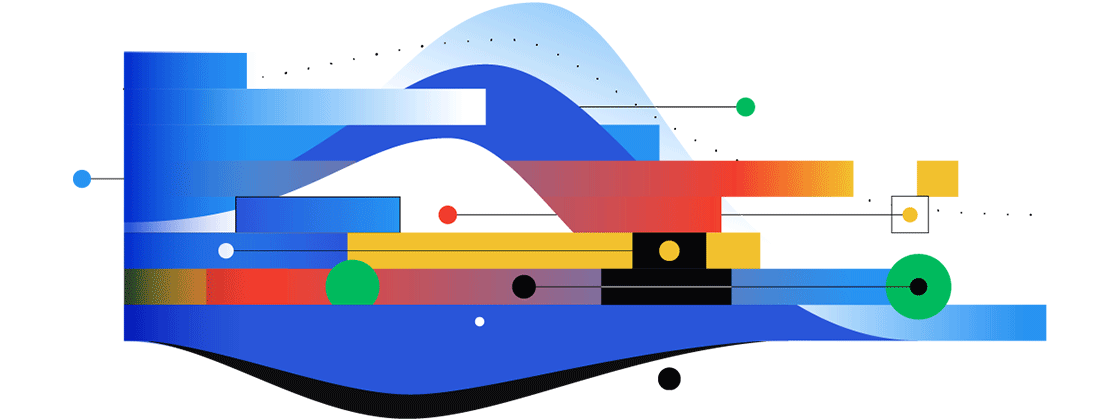
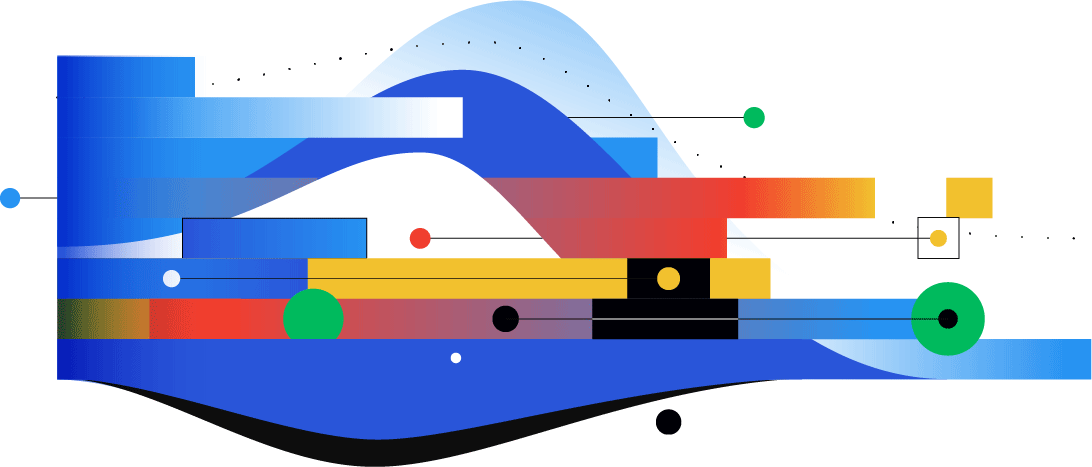
Unobtainable Numbers
Around the world, other epidemiological modelers were struggling with those new responsibilities too. The levels of asymptomatic spread were particularly difficult to accept and model. Typically, a respiratory infection gets passed through sneezing and coughing — that is, through the symptoms of the infection. SARS-CoV-2 traveled more surreptitiously. “I’m struggling actually to find other examples where there’s such a high rate of asymptomatic transmission,” said Nicholas Jewell, a biostatistician at the University of California, Berkeley.
At first, with hardly any knowledge of what the parameters for describing COVID-19 should be, researchers populated their models with numbers from the next best thing: SARS, another epidemic disease caused by a coronavirus. But using characteristics of SARS to represent the transmission dynamics of the novel coronavirus showed that the two were very, very different.
Meyers is one of the scientists who tried this. In her team’s models, even the initial numbers coming out of China “pointed out three critical things that were sort of jaw-dropping at the time,” she said. One was that, as Wu’s team had seen, the disease was spreading about twice as fast as SARS had. A significant portion of that spread was also clearly happening before people developed symptoms. And it appeared that some people could stay infectious for two weeks after they first felt symptoms, meaning that the sick and those thought to be infected would need to be quarantined for a long time.
When Meyers sent an email to the U.S. Centers for Disease Control and Prevention and others about this discovery in February, “they were like, whaaat,” she recalled — particularly about the potential levels of presymptomatic and asymptomatic transmission. “Everybody basically said, ‘Oh, you must be wrong.’ But then — I want to say within a few days — all of a sudden there was all this data coming crashing in from China, where we were like, ‘Oh my God, this is really, really bad. This thing has the makings for a pandemic that we won’t be able to control.’”
Meanwhile, other experts were seeing portents of further challenges.
Sam Abbott, an infectious disease modeler at the London School of Hygiene and Tropical Medicine, obtained his doctorate in the fall of 2019 and had just started his new research job on January 6, 2020, developing statistical methods for real-time outbreak analytics. His first project was supposed to focus on an outbreak of cholera in Yemen — but just six days later, he found himself working on COVID-19.
Abbott was accustomed to using certain statistical techniques to estimate factors governing the transmission of diseases. But he and his colleagues gradually came to a troubling realization: The accumulating evidence for asymptomatic and presymptomatic transmission of COVID-19 meant that those techniques would not always work. For example, the effective reproduction number of a disease is the average number of individuals infected by a single case at a given time during an outbreak (over time, this number can change). But if many of the cases of infection were virtually invisible, the researchers discovered, their methods for inferring the reproduction number became unreliable.
The problem didn’t jump out to the scientists immediately because “it’s quite a subtle issue,” Abbott said. “It took a while of just thinking about it and people sending graphs to each other.” The implication of the discovery, though, was that at least in some contexts, the researchers would need entirely new approaches to work out the needed variables and build useful models.
Still, even with imperfect models and incomplete data, it was clear that SARS-CoV-2 was crossing borders rapidly and that the spread was getting out of control. When the disease rampaged through a nursing home in Washington state in February, marking the beginning of the crisis in the U.S., “that was the moment when we kind of knew that we just had to drop everything that we were doing,” said Katelyn Gostic, a postdoctoral researcher in ecology at the University of Chicago who studies epidemiological problems. Screening travelers had proved entirely ineffective against this virus, which suddenly seemed to be everywhere, leaving scientists “just trying to put out the fire right in front of us,” Larremore said.
It fell to the WHO to officially pronounce COVID-19 a pandemic in March 2020. Modeling work had played one of its first crucial roles: establishing that there was a threat — here, now, everywhere — and filling in details about the nature of that threat.
And once that happened, with potentially millions of lives in the balance, policymakers and the public started demanding more answers from those models — answers about what would happen next, and when, and how society should respond — answers that the models were often in no position to provide, at least not in the form that people so desperately wanted.
Not a Crystal Ball
Because epidemiological models make statements about the future, it’s tempting to liken them to weather forecasts — but it’s also deeply wrong. The two are in no way ever comparable, as scientists who work with the models are quick to emphasize. Yet the mistaken belief that they can make similar kinds of predictions is often at the heart of public tension over modeling’s “failures.”
The quality of a weather forecast rests on how accurately and reliably it predicts whether there will be a storm tomorrow (and how long it will last and how much it might rain), despite a thousand meteorological uncertainties. But when meteorologists forecast a hurricane’s path, the decisions of people in the region to either evacuate or stay put don’t affect where the hurricane goes or how strong it will be.
In contrast, people’s actions have a direct impact on disease transmission. The additional level of uncertainty about how people will respond to the threat complicates the feedback loop between human behavior, modeling outcomes and the dynamics of an outbreak.
That’s why scientists — not just in epidemiology, but in physics, ecology, climatology, economics and every other field — don’t build models as oracles of the future. For them, a model “is just a way of understanding a particular process or a particular question we’re interested in,” Kucharski said, “and working through the logical implications of our assumptions.” Many epidemiological researchers consider gaining useful insights into a disease and its transmission to be the most crucial purpose of modeling.
Goldenfeld blames the public’s misunderstanding of models for some of the scorn hurled at his group’s reopening work for the University of Illinois. “The purpose of our model was to see if [a given intervention] could work. It wasn’t to predict on November the 17th of 2020, you’ll have 234 cases,” he said. “The point is to understand what is the trend, what is the qualitative take-home message you get from this. That’s the only thing you can reasonably expect.”
But that distinction can easily get lost, particularly when a model’s outputs are numbers that can sound deceptively precise.
At their core, all models are simplified (though not necessarily simple) representations of a system — in this case, the spread of a virus through a population. They take certain measurable features of that system as inputs: the length of the virus’s incubation period, how long people stay infectious, the number of deaths that occur for every case, and so on. Algorithms in the model relate these inputs to one another and to other factors, manipulate them appropriately, and provide an output that represents some consequent behavior of the system — the virus’s spread as reflected in the number of cases, hospitalizations, deaths or some other indicator.
To understand pandemics and other disease outbreaks, scientists turn to two well-established approaches to quantitative epidemiological modeling. Each approach has its uses and limitations, and each operates better at different scales with different kinds of data. Today, epidemiologists usually use both of them to various degrees, so their models fall on a spectrum between the two.
At one end of the spectrum are disease models that split a population into “compartments” based on whether they are susceptible, infected or recovered (S, I or R) and use systems of differential equations to describe how people might move from one compartment to another. Because COVID-19 has such a long incubation period — someone might be infected with the virus for several days before becoming capable of transmitting it to others — its models also need to include an “exposed” compartment (E). These SEIR models generally assume that populations are fairly homogeneous, that people mix relatively evenly, and that everyone is equally susceptible to the virus.
Samuel Velasco/Quanta Magazine
But of course that’s not actually true: Someone’s age, occupation, medical history, location and other characteristics can all bear on a virus’s effects and the chances of transmitting it. The most bare-bones SEIR models can therefore represent only a limited suite of behaviors. To model more complex or specialized situations — like a university reopening plan, which requires mapping out processes like disease testing and contact tracing, as well as detailed patterns of interactions among groups of students, faculty and staff — researchers have to move away from simple averages.
To achieve that, they can embellish their SEIR models by adding more structure into how the disease spreads. Meyers’ group, for instance, built a massive model, composed of interconnected SEIR models, that represented the viral transmission dynamics within dozens of subpopulations in each of the 217 largest U.S. cities; they even accounted for the movements of people between those cities.
As researchers add these layers of detail, they move to the other end of the epidemiological modeling spectrum: toward agent-based models, which don’t average across groups of people but rather simulate individuals, including their interactions, daily activities and how the virus itself might affect them if they get infected. (The model that Goldenfeld and Maslov developed for the University of Illinois was agent-based.) This granularity allows the model to capture some of the inherent heterogeneity and randomness that get abstracted away in the simplest SEIR models — but at a cost: Scientists need to gather more data, make more assumptions, and manage much higher levels of uncertainty in the models. Because of that burden, they usually don’t aspire to that level of detail unless their research question absolutely requires it.
These kinds of models can reveal a lot about diseases by inferring parameters such as their reproduction number, their incubation period, or their degree of asymptomatic spread. But they can also do much more. For COVID-19, such models have suggested that people who are asymptomatic or have mild symptoms are only about half as contagious as the more obviously sick, but are responsible for approximately 80% of documented infections. Similarly, they have shown that young children are about half as likely as adults to get infected if exposed to someone with COVID-19, but that this susceptibility increases rapidly for children over the age of 10. The list of examples goes on.
Samuel Velasco/Quanta Magazine
With those values in hand, the models can also project portfolios of what-ifs: If a city lifts lockdown measures on stores but not restaurants, what might happen to case counts? How effective does a contact-tracing program need to be to allow a school to reopen, or to ensure that the local hospital system doesn’t get overwhelmed? Models can also assist with more practical and immediate decision support: If disease transmission looks a certain way, how much personal protective equipment should a hospital purchase, and how should the dissemination of vaccines be prioritized?
But those are projections — not predictions. They are contingent on assumptions that can change overnight, and as a result they are rife with uncertainty.
To be sure, these projected futures can still lead to incredibly useful insights. From models of how COVID-19 spreads, for instance, Kucharski and his team learned that contact tracing alone would not be enough to contain the disease; additional measures would have to support it. Other research showed how important testing was, not just for measuring the scope of the epidemic but as an actual mitigation measure: Models by Larremore and others indicated that less sensitive but faster tests would be far preferable to slightly slower but more accurate ones. Still other modeling work has been instrumental in deciding how many additional staff and beds a hospital might need.
Even so, it’s easy for the distinction between projections and predictions to get lost when people are desperate for answers. Researchers have seen that happen in almost every pandemic, and they’ve seen it happen during this one — starting with the very first projection modelers usually decide to publish: a worst-case scenario.
A Worst-Case Reaction
In mid-March, when a research team at Imperial College London announced that their agent-based model had projected up to half a million deaths from COVID-19 in the United Kingdom and 2.2 million deaths in the U.S. over the course of the epidemic, it was an estimate of the death toll that could occur if society did literally nothing in response. It was a hypothetical, a consciously unrealistic projection that could begin to map the terrain of what might happen. It was never supposed to be a prediction.
Why, then, did Imperial College publish it? Partly because it helped to establish a baseline for evaluating how well any intervention might be working. But also because it prompted action around the globe: It was one of the models that were instrumental in getting countries to lock down and consider other drastic measures. “It’s a valid use of models,” said Matthew Ferrari, a quantitative epidemiologist at Pennsylvania State University, “to raise alarms and initiate action, like state lockdowns and national mask mandates and stuff, that might prevent that future from coming to pass.”
But that galvanizing effect comes with a risk of misinterpretation. Justin Lessler, an epidemiologist at Johns Hopkins University, says that when he discusses scenario models for pandemics with nonexperts, he tries to emphasize that the answers are often right only within an order of magnitude. “I’m always very clear with people,” he said. “It always gets interpreted as a proper forecast. But at least you say it.”
So in the early spring, when the mortality statistics in the U.K. seemed less grim than the Imperial College model’s figures, the discrepancy led to accusations of sensationalism and some public distrust.
“People … said the model’s wrong because the scenarios you explored aren’t what happened,” said James McCaw, a mathematical biologist and epidemiologist at the University of Melbourne in Australia. “That’s because the scenarios terrified us, and so we responded to avoid it. Which is not that the model’s wrong, it’s that we responded.”
This kind of misperception isn’t new to epidemiology. During the 2014 Ebola epidemic in West Africa, U.S. CDC modelers published the upper bounds on their projections for the size of the outbreak. They suggested there could be around 1 million deaths, though in the final tabulation, fewer than 12,000 people died. The groups who reported those estimates had to weather intense criticism for supposedly sensationalizing the outbreak. But the critiques ignored the fact that this was only a worst-case scenario, one that frightened people enough to prompt responses that forestalled the worst-case reality.
McCaw says that when his team and colleagues used worst-case-scenario estimates to model their own situation with COVID-19 in Australia, they began to see “some of the very scary numbers that came out of that,” and the model played a major part in the country’s decision to close its borders. In fact, Australia made that call early on and quickly enforced strict physical distancing measures, mandating that people not leave their home except to take care of essential activities.
In hindsight, Australia benefited greatly from that smart choice: The country saw an outbreak that was significantly smaller and easier to control than what unfolded in many other nations that took longer to act. So did Taiwan, South Korea, Singapore, New Zealand and other regions, particularly those that applied lessons learned during the SARS and MERS epidemics to mount quick and effective responses. But at the time, “various places around the world criticized Australia for doing that,” McCaw said. Only later, when the devastation elsewhere became apparent, did some of the criticism die down.
“You end up with people not really trusting the models because they say, ‘We’re locking down a country of 5 million people for the sake of 100 cases,’ which seems ridiculous on the surface,” said Kevin Ross, who directs a collaborative effort between New Zealand’s health and academic sectors. “But it’s not for the sake of 100 cases, it’s for the sake of avoiding 100,000 cases.”
A Story They Wanted to Believe
Unfortunately, conflation of projection with prediction wasn’t limited to worst-case scenarios, and the misinterpretations weren’t limited to public opinion. Other modeling estimates were taken the wrong way, too — and by people with decision-making power.
It perhaps didn’t help that there was an over-proliferation of models from researchers far afield of epidemiology: an explosion of preprints from physicists, economists, statisticians and others who had their own extensive experience with complex modeling and wanted to help end the pandemic. “I think everybody who had a spreadsheet and had heard the words ‘S,’ ‘I’ and ‘R’ felt they should make a model,” Lessler said. “I don’t want to say none of them did a good job, but for the most part, it’s not so much about the math and the technical stuff. It’s more about understanding where you can go wrong in the assumptions.”
Scarpino agreed. “We have somehow managed to do this for every single pandemic and outbreak that ever happens,” he said of well-intended scientists entering the epidemiological modeling arena — and while that can be helpful, it also runs the risk that they might “just reinvent broken wheels.”
One of the first models to capture the ear of the White House was a statistical model published by the University of Washington’s Institute for Health Metrics and Evaluation (IHME). The IHME’s primary expertise is in analyzing the efficacy of health systems and interventions, but the organization wasn’t particularly experienced in epidemiological forecasting or infectious disease modeling as such.
As a result, their model technically qualified as epidemiological, but it didn’t take into account the virus’s mechanism of transmission or other key characteristics of the epidemic. Instead, it fit a curve to mortality data in the U.S. based on several basic premises: that the curve would take the same general shape as it did in China and Italy, where the infection rate was already declining; that people would generally comply with government-level policies and mandates; and that social distancing and other interventions would have the same impact as in China.
A key caveat, though, was that the IHME’s model relied entirely on those assumptions. If any of those conditions changed or weren’t quite right in the first place, the model’s outputs would no longer be relevant.
And many of the model’s assumptions were already not holding. People’s behavior wasn’t coupled to the implemented policies: They got scared and started social distancing long before governors announced stay-at-home orders. But “stay at home” and “social distancing” by U.S. standards also looked nothing like what was being enforced in China. While the epidemic in China followed a simple rise-and-fall progression, the U.S. was hitting a second peak before its first ended, forming a “double S” shape. Unguided by any underlying biological mechanism, the model had no way to account for that changing dynamic. As a result, its estimates fell overwhelmingly short of reality.
“Some of the original forecasts from the IHME group were using a method that had been thoroughly debunked before I probably was even born,” Scarpino said.
That might not have mattered in the long run if the model had been used properly. Typically, epidemiologists use a statistical model like the IHME’s as part of what’s known as an ensemble forecast. (Despite its name, an ensemble forecast is really like a model built from other models, and it too offers only projections, not predictions.) The IHME model’s results would be averaged and weighed mathematically with the outputs of dozens of other epidemiological models, each with its own shortcomings, to achieve the modeling equivalent of the “wisdom of the crowd.” One model in an ensemble might be better at handling unlikely events, another might excel at representing transmission when the caseload is higher, and so on; some models might be statistical, others might be SEIR types that stratify populations in different ways, still others might be agent-based.
Samuel Velasco/Quanta Magazine; source: https://viz.covid19forecasthub.org
“Consensus is not always what you’re after. You don’t want groupthink,” McCaw said. With so much uncertainty in the science and data, “it’s good to have multiple models in different perspectives.”
Comparisons among multiple models can also substitute to a degree for vetting their quality through peer review. “You can’t do peer review fast enough to help in an epidemic,” Goldenfeld said. “So you have to do peer review not sequentially … but in parallel.”
Unfortunately, Lessler said, “it took longer than it should” to get those ensembles up and running in the U.S. The lack of a coordinated national response may have been a root cause of the delay. Scientists were left to their own devices, without the resources they needed to pivot easily from their everyday work to the around-the-clock dedication that COVID-19 ended up requiring. Often, they essentially had to volunteer their time and effort without proper funding and had to establish networks of communications and collaboration as they worked. Much of the infrastructure that could have helped — a pandemic preparedness group in the White House, a centralized top-down organizational effort to connect expert modeling teams with other researchers and officials, and of course core funding — was entirely absent.
Without the ensembles, that left the IHME model, with its single perspective and other problems, as the most appealing strategic resource available to many decision-makers.
“When COVID emerged, the IHME model seemed to come out of nowhere and really got a ton of attention,” Meyers said. “It was being cited by Trump and the White House coronavirus task force, and they had a really nice, visually intuitive webpage that attracted public attention. And so I think it was really one of the most noticed and earliest forecasting models to really put forecasting on the radar in the public imagination.”
Epidemiologists grew alarmed when, in April and May, the IHME projections were used by the White House and others to say that the U.S. had passed the peak of its outbreak, and that case numbers and deaths would continue to decline. Such claims would hold true only if the U.S. stayed under lockdown.
But “people used those models [and others] to reopen their cities and justify relaxing a lot of the stay-at-home orders,” said Ellie Graeden, a researcher at the Georgetown University Center for Global Health Science and Security and the founder of a company that specializes in translating complex analysis into decision-making. “It suggested a degree of optimism that I think assuaged concern early in the event.”
Graeden thinks this made it much harder to get the public and decision-makers to heed more realistic scenarios. “It’s not that IHME was the only model out there,” she said. “It was a model that was showing people the story that they wanted to believe.”
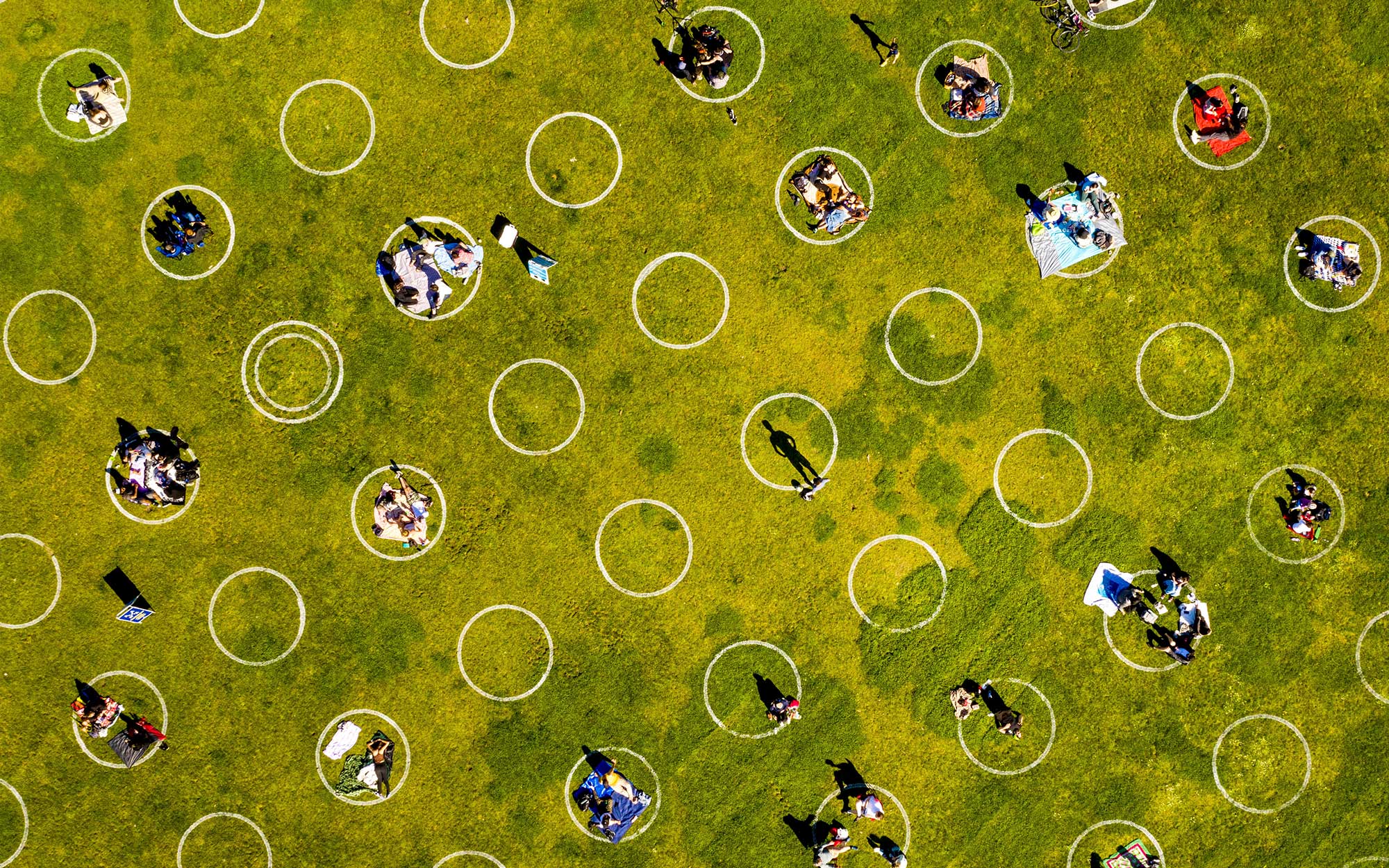
Small groups of people gathering in Dolores Park in San Francisco during the pandemic each stay within their socially distanced designated areas.
The IHME has since revised its model repeatedly, and other research teams, including Meyers’ group, have used their epidemiological experience to build on some of its core machinery and improve its projections. (Those teams have also developed their own new models from scratch.) The current version of the IHME model is one of many used in an ongoing ensemble forecasting effort run by the CDC. And the IHME has since become more transparent about its assumptions and methods — which has been crucial, given the extent to which uncertainties in those assumptions and methods can propagate through any model.
After all, even the best models are dogged by uncertainties that aren’t always easy to recognize, understand or acknowledge.
Reckoning With Uncertainty
Models that rely on fixed assumptions are not the only ones that need to be navigated with care. Even complex epidemiological models with built-in mechanisms to account for changing conditions deal in uncertainties that must be handled and communicated cautiously.
As the epidemic emerged around her in Spain, Susanna Manrubia, a systems biologist at the Spanish National Center for Biotechnology in Madrid, became increasingly concerned about how the results of various models were being publicized. “Our government was claiming, ‘We’ll be reaching the peak of the propagation by Friday,’ and then ‘No, maybe mid-next week,’” she said. “And they were all systematically wrong, as we would have expected,” because no one was paying attention to the uncertainty in the projections, which caused wild shifts with every update to the data.
“It was clear to us,” Manrubia said, “that this was not something that you could just so carelessly say.” So she set out to characterize the uncertainty rooted in the intrinsically unpredictable system that everyone was trying to model, and to determine how that uncertainty escalated throughout the modeling process.
Manrubia and her team were able to fit their models very well to past data, accurately describing the transmission dynamics of COVID-19 throughout Spain. But when they attempted to predict what would happen next, their estimates diverged considerably, sometimes in entirely contradictory ways.
Manrubia’s group was discovering a depressing reality: The peak of an epidemic could never be estimated until it happened; the same was true for the end of the epidemic. Work in other labs has similarly shown that attempting to predict plateaus in the epidemic curve over the long term is just as fruitless. One study found that researchers shouldn’t even try to estimate a peak or other landmark in a curve until the number of infections is two-thirds of the way there.
“People say, ‘I can reproduce the past; therefore, I can predict the future,’” Manrubia said. But while “these models are very illustrative of the underlying dynamics … they have no predictive power.”
The consequences of the unpredictability of those peaks have been felt. Encouraged by what seemed like downturns in the COVID-19 numbers, many regions, cities and schools reopened too early.
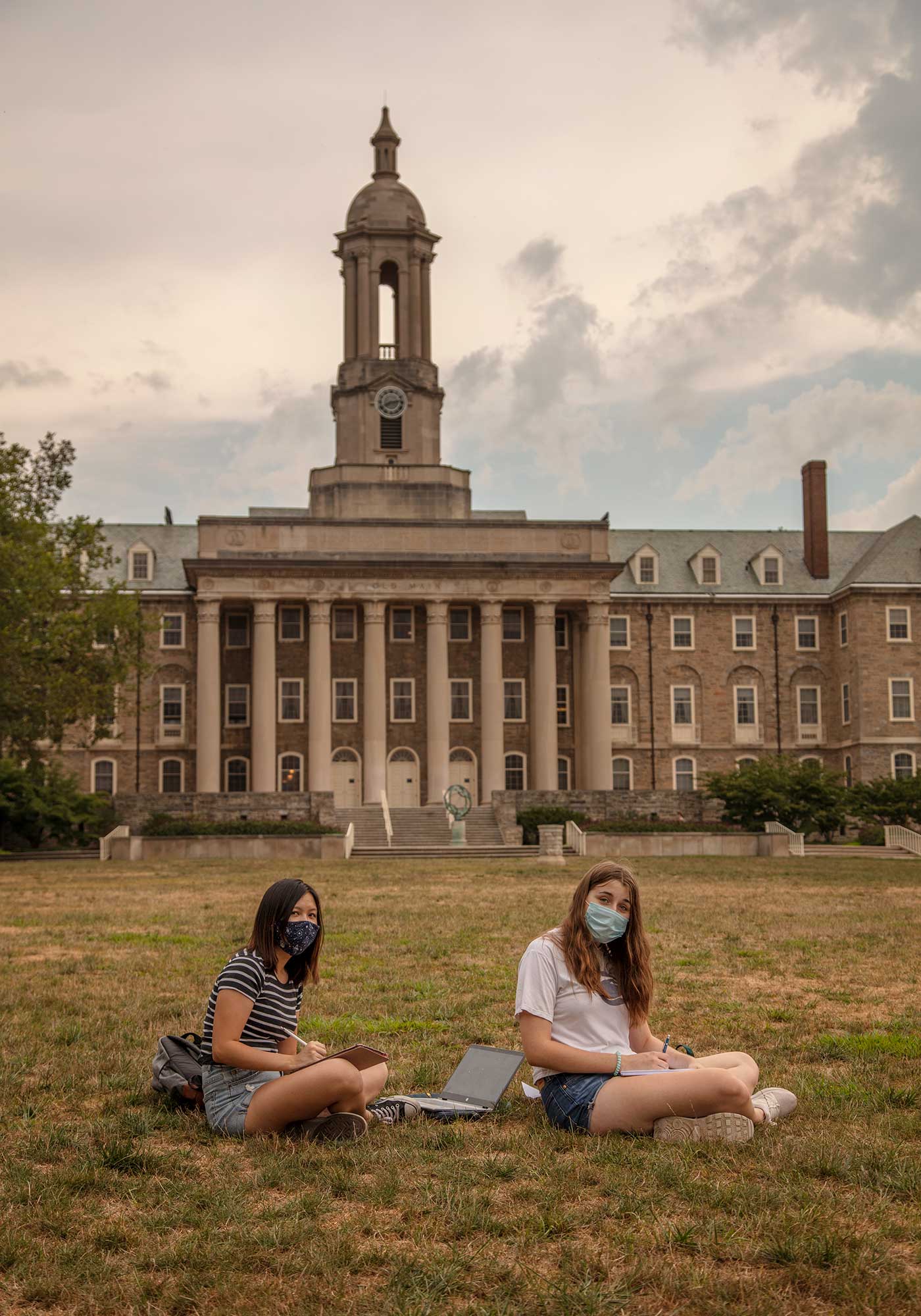
Students Michelle Vu (left) and Klaudia Bak at Pennsylvania State University, after it reopened its campus last fall. School reopening plans across the country had to adjust to unexpected behaviors and events that had the potential to cause large outbreaks.
Ferrari and his colleagues at Penn State, for instance, had to confront that possibility when they started making projections in March about what August might look like, to inform their more granular planning models for bringing students back to campus. At the time, it seemed as if the first wave of infections would be past its peak and declining by the summer, so Ferrari and the rest of the modeling team assumed that their focus should be on implementing policies to head off a second wave when the students returned for the fall.
“And then the reality was, as we got closer and closer, all of a sudden we’re in June and we’re in July, and we’re all yelling, ‘Hey, the first wave’s not going to be over,’” Ferrari said. But the reopening plans were already in motion. Students were coming back to a campus where the risk might be much greater than anticipated — which left the team scrambling to find an adequate response.
Chasing the Data
An unfortunate early lesson that COVID-19 drove home to many researchers was that their modeling tools and data resources weren’t always prepared to handle a pandemic on the fly. The biggest limitations on a model’s capabilities often aren’t in its mathematical framework but in the quality of the data it uses. “The best model could not account for our lack of knowledge about epidemiology, about the biology,” Wu said. Only good data can do that.
But gathering data on a pandemic as it happens is a challenge. “It’s just an entirely different ballgame, trying to produce estimates in real time,” Gostic said, “versus doing research in what I would describe as more of a peacetime scenario.”
“It’s a war,” McCaw agreed: one waged against chaos, against inaccuracies, against inconsistencies, against getting completely and utterly overwhelmed. “It’s really hard to get the right information.”
A key number that epidemiological modelers want to know when collecting data, for instance, is the total number of infections. But that’s an unobservable quantity: Some people never visit a doctor, often because they have mild symptoms or none at all. Others want to get tested to confirm infections but can’t because of an unavailability of tests or lack of testing infrastructure. And even among those who do get tested, there are false positives and false negatives to consider. Looking at the number of reported cases is the next best option, but it’s just the tip of the iceberg.
Gathering even that data in a timely way for COVID-19 was often nearly impossible early on. “I grew up in the information age, and so I guess I naively assumed at the start of this pandemic that state departments of public health would have some sort of button, and they could just press that button and data from hospitals around the state would just automatically be routed to some database,” Gostic said. “But it turns out that that button doesn’t exist.”
Scientists who had hoped to immediately start building useful models instead spent most of February and early March just trying to gain access to data. They spent weeks cold-calling and emailing hospitals, departments of public health, other branches of government and consulting companies — anyone they could think of. Researchers had to sort through texts, faxes, case reports in foreign languages and whatever else they could get their hands on, all the while worrying about where that data was coming from and how accurate it was.
It’s been “a real disappointment and a surprise,” said John Drake, an ecologist at the University of Georgia, “that as a country or globally, we’ve done such a poor job collecting data on this epidemic. … I genuinely thought that there would be a government response that would be effective and coordinated, and we haven’t had that.”
“None of us, I think, were prepared for the inconsistent data collection,” he added.
In those early days of the epidemic, case data in the U.S. and other regions was so unreliable and unrepresentative that it often became unusable. Case counts were missing large numbers of asymptomatic and mildly symptomatic infections. Testing and reporting were so scarce and inconsistent that it distorted the numbers that researchers obtained. Pinning those numbers down in real time was further complicated by the lag between when a person got infected and when they showed up in the reported case data. Even the very definition of a “case” of COVID-19 changed over time: At first, an infection was only considered an official case if a person had traveled to Wuhan, exhibited particular symptoms, and then tested positive (the tests were different then, too). But as weeks and then months passed, the criteria kept expanding to reflect new knowledge of the disease.
For some researchers, these problems meant turning to data on hospitalizations and deaths from COVID-19. The recordkeeping for those numbers had its own shortcomings, but in some ways it was more reliable. Yet that data captured still less of the full picture of the pandemic.
“Models aren’t a substitute for data,” Kucharski said.
It wasn’t until late April or May, with the establishment of more comprehensive testing (as well as more reliable pipelines for case data), that some scientists started feeling comfortable using it. Others tried to account for the issues with case data by applying various statistical techniques to translate those numbers into something more representative of reality. As always, there was no right answer, no obvious best path.
Because of these complications, it took months to pin down good estimates for some of the key variables describing COVID-19. During that time, for example, estimates of the proportion of asymptomatic cases jumped from 20% to 50%, then back down to 30%.
Modeling groups also put out diverse estimates of the infection fatality ratio — the number of people who die for every person who gets infected, an important parameter for estimating a potential death toll. Every aspect of the calculation of that figure had huge uncertainties and variability — including that the number itself can change over time, and that it differs based on the demographics of a population.
Unfortunately, the infection fatality ratio is also a number that has been heavily politicized in a way that demonstrates “an abuse of models,” Larremore said. Factions pushing for earlier reopening, for instance, emphasized the lower estimates while disregarding the related epidemiological considerations. “People have their conclusions set a priori, and then there’s a menu of possible models, and they can look at the one that supports their conclusions.”
Part of what built researchers’ confidence in the values they were getting was the emergence of special data sets that they could use as something like an experimental control in all the chaos. For example, one of the largest known COVID-19 outbreaks in February outside of China occurred on the Diamond Princess cruise ship, docked in quarantine off the coast of Japan, where more than 700 people were infected. Over time, scientists reconstructed practically a play-by-play of who was infected when and by whom; it was as close to a case study of an outbreak as they were likely to get. The Diamond Princess event, along with similar situations where the surveillance of populations captured the spread of the disease in extraordinary detail, told researchers what they needed to know to reduce the uncertainty in their estimates of the infection fatality ratio. That in turn helped improve their models’ projections of the total number of deaths to expect.
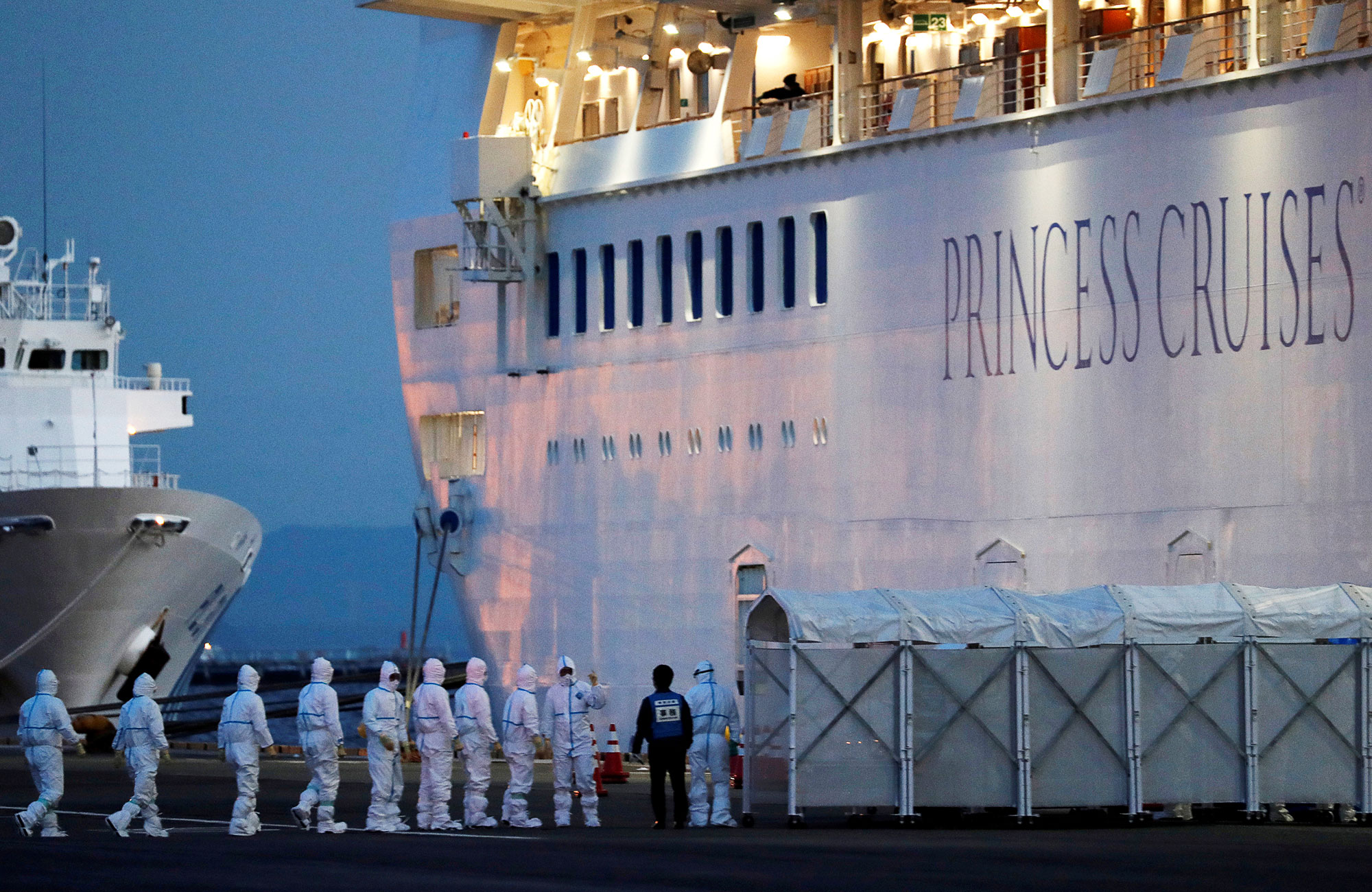
Detailed information about how the coronavirus was transmitted among hundreds of passengers on the Diamond Princess cruise ship was instrumental in helping researchers estimate the values of key epidemiological variables for COVID-19.
Some of the most comprehensive data came in the summer months and beyond, as testing became more prevalent, and as researchers designed serology studies to test people in a given population for antibodies. Those efforts gave a clearer snapshot of the total number of infections, and of how infection related to other factors.
But these parameters are ever-moving targets. In the U.S., for instance, researchers observed a drop in the infection fatality ratio as hospitals improved their treatments for the disease, and as changing behavior patterns in the population led to a higher proportion of infections among young people, who had a better chance of recovering. Only constant updates with high-quality data can help researchers keep up with these changes.
Obtaining good data on COVID-19 continues to be a problem, however, not just because of shortcomings in the data collection process but because of intrinsic characteristics of the virus that affect the data. Even the reproduction number has proved trickier to estimate than expected: Because COVID-19 mostly spreads through random, infrequent superspreader events, a simple average value for how quickly it’s transmitted isn’t as useful. Moreover, during past epidemics, modelers could estimate changes in the reproduction number over time from data about the onset of symptoms. But since so many COVID-19 infections occur asymptomatically or presymptomatically, symptom onset curves can be misleading. Instead, modelers need curves based on infection data — which for COVID-19 can only be inferred. This messiness makes it difficult to look back and analyze which interventions have worked best against the disease, or to draw other conclusions about apparent correlations.
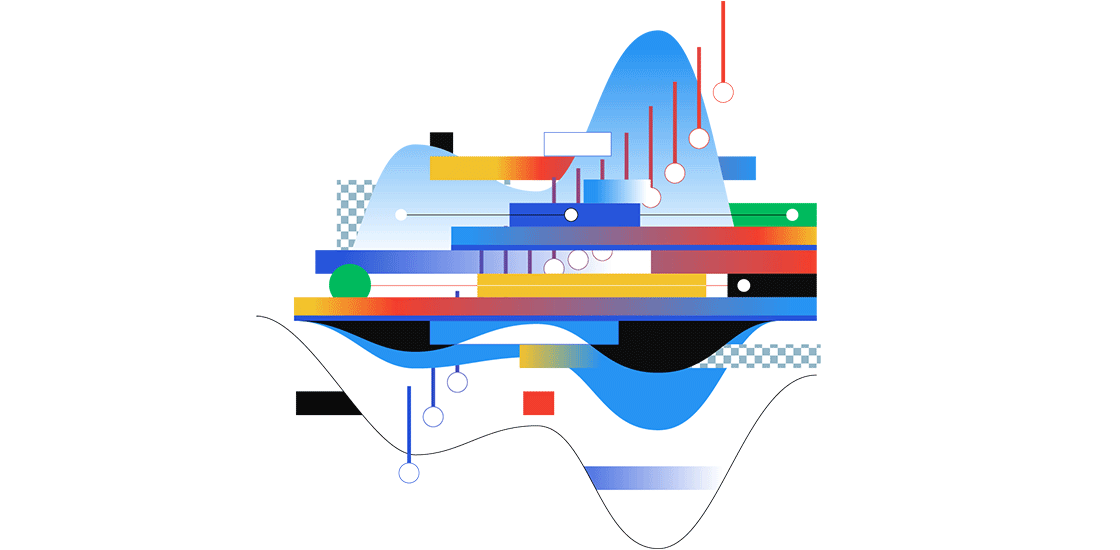
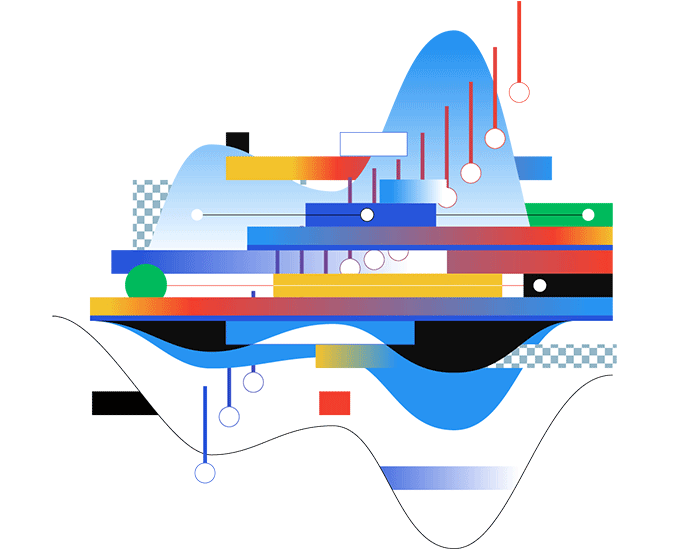
The Biggest Problem Is Us
But by far, the biggest source of uncertainty in COVID-19 models is not how the virus behaves, but how people do. Getting that X-factor at least somewhat right is crucial, given just how much people’s actions drive disease transmission. But people are capricious and difficult to understand; they don’t always act rationally — and certainly not predictably.
“Modeling humans is really hard,” Graeden said. “Human behavior is idiosyncratic. It’s culture-specific,” with differences that show up not just between nations or demographics but between neighborhoods. Scarpino echoed that idea: “You walk across the street and it’s a different transmission dynamic, almost,” he said.
Ferrari and his colleagues have seen just that at Penn State. Since the fall, they’ve repeatedly conducted antibody tests on both university students and people who live and work near campus. They found that even though the outbreak infected 20%-30% of the 35,000 students, the surrounding community had very little exposure to COVID-19. Despite their proximity, the students and the townsfolk “really did experience completely different epidemic settings,” Ferrari said.
Those differences weren’t limited to behavioral or cultural practices but extended to systemic considerations, like the ability to work from home and access to resources and care. “I think most people, given the opportunity, would exhibit good individual behavior,” Ferrari said. “But many people can’t, because you first need the infrastructure to be able to do so. And I think those are the kinds of insights that we’re slowly moving towards trying to understand.”
A further complication is that past sociological studies of human behavior no longer apply: During a pandemic, people simply aren’t going to behave as they normally do.
“One of the big sort of ‘oh no’ moments,” Meyers said, “was when we realized that everything we’d been assuming about human contact patterns and human mobility patterns was basically thrown out the window when we locked down cities and sheltered in place. We didn’t have the data. We didn’t have the pre-baked models that captured this new reality that we were living in.”
Unfortunately, top-down regulations can’t be used as proxies for people’s actual behaviors. Anonymized data about people’s movements — from cell phones and GPS-based services — has shown that people mostly stopped moving around early in the pandemic, independently of whether lockdowns were in place in their region; people were scared, so they stayed at home. Meanwhile, interventions like mask mandates and bans on indoor dining were instituted but not always enforced, and people gradually moved around and interacted more as the months wore on, even as the number of deaths per day rose to unprecedented heights.
Those kinds of behaviors can significantly complicate the shapes of epidemic curves. But knowledge of those behaviors can also illuminate the deviations between what researchers observed and what they expected. For example, the modeling for the spread of COVID-19 through nursing homes and long-term care facilities didn’t initially match the observed data. Eventually, scientists realized that they also had to take into account the movement of staff members between facilities. But it took time to pinpoint that behavioral factor because such specific movements are usually abstracted away in simpler models.
“Confronting the link between behavior and transmission is difficult,” said Joshua Weitz, a biologist at the Georgia Institute of Technology, “but it has to be prioritized to improve forecasting, scenario evaluation, and ultimately to design campaigns to more effectively mitigate and control spread.”
This realization led researchers to pursue data from cell phones and other sources, to design comprehensive surveys about interactions and other activities outside and within households, and to integrate that information into epidemiological models at a massive scale. “We hadn’t actually really developed the technology to do that [before],” Meyers said, because there had been no urgent call for that data (or no access to it) before COVID-19. A new methodology was needed, along with new ways to assess the quality and representativeness of the data, which was often supplied by private companies not subject to the same scrutiny as other epidemiological data sources. “All of that we’ve developed in the last few months,” she said.
Uncertain Communications
These different types of uncertainty add up and have consequences down the line. Because small uncertainties can have exponentially bigger effects, “it’s a little bit like chaos,” Goldenfeld said. But communicating that uncertainty to decision-makers in a way that’s still useful and effective has proved a particularly difficult task.
“Decision-makers want answers,” Graeden said, but “a model cannot produce an answer. And it shouldn’t. It can get close. It can be suggestive.” To her, the pandemic has only highlighted a long-standing communications challenge. “There’s actually a culture clash here, where fundamentally the communication always will be broken, because the scientist has been trained not to give an answer, and the decision-maker’s only job is to provide one.”
Abbott recalls getting feedback from decision-makers about having too much uncertainty in some of his modeling work. “I’m not sure what the happy balance is,” he said. “You need what actually happens to be encapsulated by your estimates going forward; otherwise people lose confidence. But equally, if you’re too uncertain, people can’t make any decisions off your estimates.” When asked what he’d done since getting the feedback about reducing uncertainty, “I accidentally added more,” he said, a bit sheepishly.
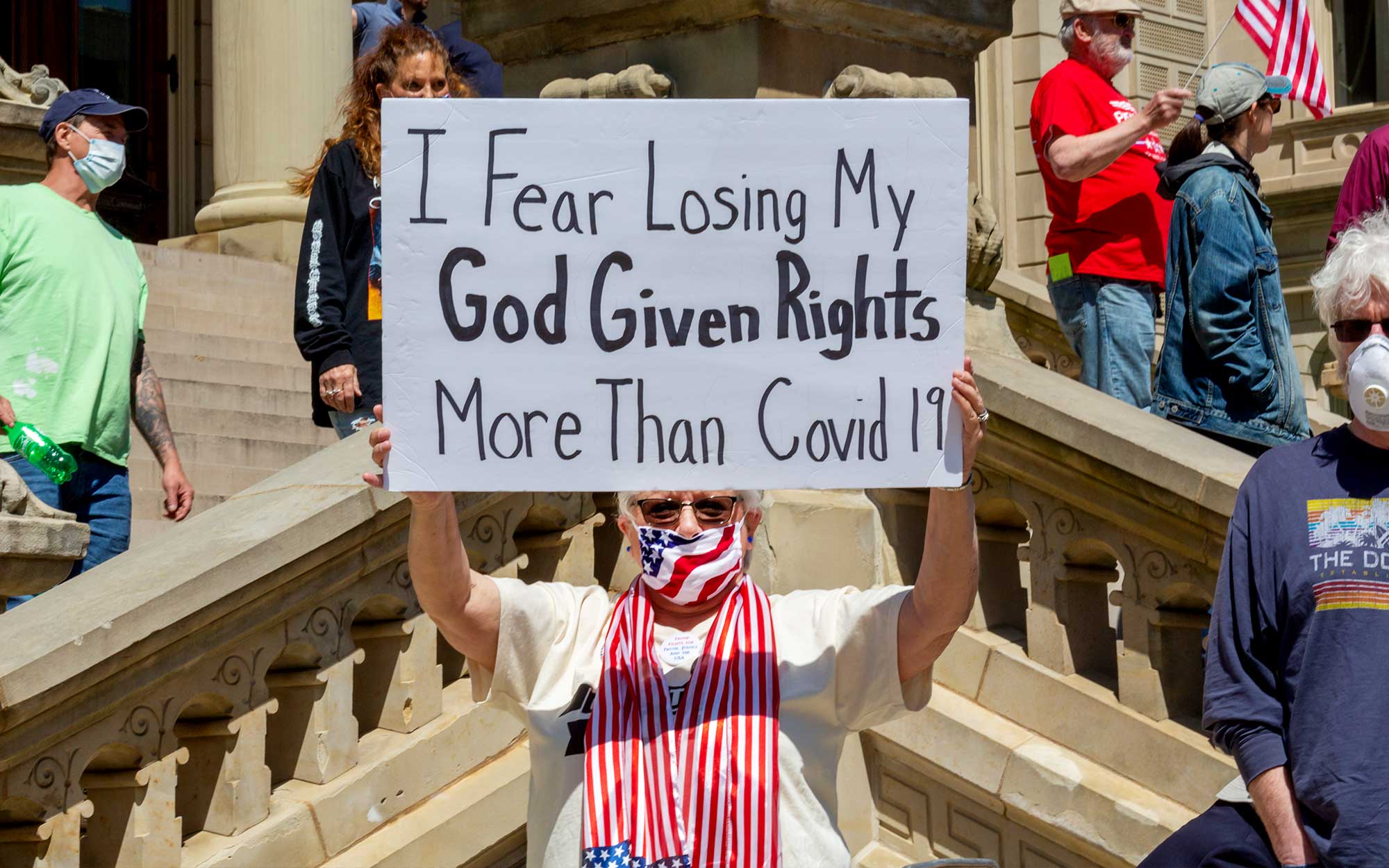
A group protests against lockdown orders from the governor of Michigan in May, in defiance of recommendations for mask-wearing and social distancing. People’s behaviors have often contradicted expectations during the pandemic.
One way researchers and decision-makers have tried to bridge that culture gap is to focus on qualitative narratives that emerge from all the models, rather than specific quantitative outcomes. Just knowing something as simple as whether the epidemic is growing or shrinking at a given time can be immensely helpful, even if different models spit out very different numbers to reflect it. The trend can be used to analyze which interventions have been most effective in various contexts, and to suggest which combinations of interventions might be best to try going forward.
“Trends are more robust than precise numbers, and can be used to plan responses,” Goldenfeld said. “Instead of saying, ‘Can you predict for me how many hospital beds am I going to need?’ what you can predict is, ‘What is the probability in the next, say, three weeks that the hospital capacity in this region is going to be exceeded … and by how much?’”
Relying on multiple models also allows researchers and decision-makers to address a variety of assumptions and approaches to bolster confidence in whatever conclusion they reach. Kucharski explained that if two completely different models both conclude that some level of contact tracing isn’t enough to control an outbreak, “then the difference in structure and the differences in precise estimates are less relevant than the fact that they both come to the same answer about that question.”
Even the uncertainty itself can be informative. In December, officials at Penn State, including Ferrari and his team, initially settled on reopening their campus in mid-January. But as 2020 drew to a close, they decided to push that plan back a month: Students will now be returning to campus on February 15. Because of factors such as the rise in levels of transmission in the surrounding county, there was “a real concern that we just wouldn’t be able to maintain operations at the level that was necessary to bring students back,” Ferrari said.
But the decision was also based on uncertainties in the projections of a model that Ferrari and his colleagues had been looking to for guidance — the CDC’s ensemble forecast, which aggregates the results of dozens of individual models. In December, the bounds on the uncertainties were so wide that “we didn’t really quite know what January was going to look like,” he said.
He and his team also noticed that many of the individual models in the ensemble were under-predicting the number of cases that would arise. “For the three to four weeks before that decision got made, we were seeing in reality worse outcomes than the models were projecting,” Ferrari said. “So that gave us pause and made us think that really, the coming three to four weeks are more likely to be on the pessimistic side of that confidence bound. And we just really couldn’t accept that.”
The trustworthiness of the models isn’t the only consideration in setting policy. According to McCaw, it also helps to have cultivated relationships with government officials and other policy-setters. He and members of his team have spent 15 years doing this in Australia, mostly through discussions about how best to respond to flu epidemics. “We’ve had hundreds and hundreds of meetings now,” he said. “We learn each other’s styles, we learn each other’s quirks, and we have a lot of trust.” Those long-term relationships have helped him figure out how to explain his work in a way that not only makes sense to whoever he’s interacting with, but also allows them to explain it to other policy leaders.
In line with that goal, over the past five years and more, McCaw and his colleagues have conducted workshops and programs to figure out how best to visualize and communicate their modeling. (Tables of numbers work surprisingly well. Though they “can feel a bit overwhelming and busy,” according to McCaw, “they’re a high-dimensional visualization of something, so they’re actually quite powerful.” Heat maps are less successful for anything other than geographical data. Graphs have their uses, but they need to be directed at a particular policy question.) Most importantly, the Australian team learned that “shying away from uncertainty is a disaster,” McCaw said. “So we embraced that.”
Other researchers have gone straight to social media instead, posting their preprints and models on Twitter to inform the public directly and to gain access to academics and government officials through less formal channels.
These forms of communication remain difficult and time-consuming, and many researchers have had to learn about them on the fly as the pandemic progressed. “It’s really made me wish that there were more communication lessons in STEM education,” said Kate Bubar, a graduate student in Larremore’s lab at the University of Colorado, Boulder who started pursuing her doctorate during the COVID-19 crisis.
The exchanges with policy-setters also benefit the scientists by illuminating decision-making processes, which can seem opaque when they go beyond the models’ math to consider other factors.
When case numbers at Penn State shot up after its reopening, Ferrari and others at first urged university officials to close the campus again immediately. But then, he recalls, the head of university housing told him, “Matt, do you understand what ‘shut it all down, send them all home’ means?” He pointed out that the school’s 40,000 students would not suddenly disperse; 30,000 of them would likely stay in the apartments they had rented. Moreover, the logistics of getting everyone off campus were intimidatingly hard and could even worsen the risk of spreading COVID-19.
“And I think each one of us went through cases like that,” Ferrari said, “where we got to the end of a really impassioned discussion and said, ‘OK, you know, I’m going to stand down because now that I can see the case from your perspective, I recognize all of the things that I was glossing over.’”
How Models Can Help Next
Epidemiological models will continue to be crucial in what everyone hopes will be the end stages of the pandemic, particularly as researchers look into the next big questions, such as how to prioritize the dissemination of vaccines. A common way to do so for the flu, for instance, is to target vaccines at children, which indirectly protects the rest of the population because kids are a major node in the network of flu transmission (though they do not seem to be for COVID-19).
Larremore and Bubar have been part of the ongoing effort to model what that should look like for COVID-19. Long before any vaccines were available, they considered an extensive list of uncertainties: about the disease dynamics, about whether a vaccine would block disease transmission, about how long immunity might last, and other factors. A big question was how effective a vaccine needed to be, and in what way that efficacy would manifest: If a vaccine was 50% effective, did that mean it worked perfectly but only in 5 out of 10 people, or that it cut everyone’s chances of infection in half?
They quickly found that in setting COVID-19 vaccination strategies, it’s necessary to choose between the goals of reducing deaths and reducing transmission. If they wanted to minimize mortality, direct vaccination of the elderly was the way to go, regardless of vaccine efficacy and other what-ifs. But if the goal was instead to reduce transmission (in places with large numbers of essential workers who couldn’t stay in lockdown, for instance), then adults between the ages of 20 and 50 should be vaccinated first instead: Their behaviors and interactions made them more of a hub for further spread. In that case, however, there would also be slight differences in allocation based on the efficacy of different vaccines, population demographics and contact patterns. “We got different results for Brazil, Belgium, Zimbabwe and the U.S.,” Larremore said.
Now that multiple vaccines have been approved in various countries around the world, researchers have been able to refine those models. But they’ve also had to expand on their work to account for new events that are shifting priorities again. One is the emergence of new SARS-CoV-2 mutations that have raised the transmission rate in many regions. Another is that the rollout of vaccines is happening much, much more slowly than had been anticipated.
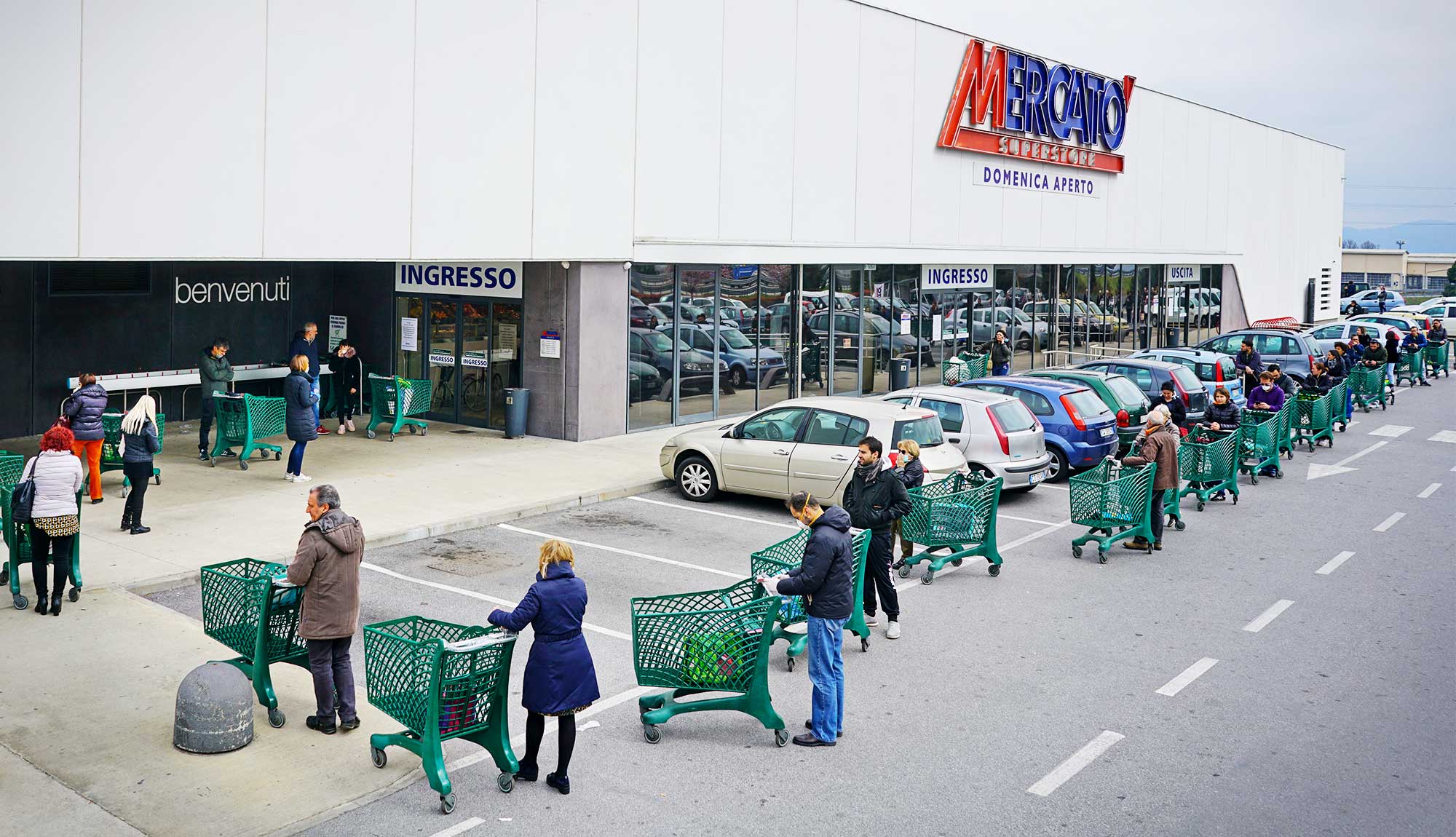
People in Milan, Italy, wait in long lines — a socially distanced six-feet apart — to go grocery shopping.
Still, to Larremore, the basic calculus stays the same. “It’s a race,” he said. “It’s racing against the virus itself that’s hopping from person to person.” And so, “as you roll the vaccine out more and more slowly, it’s going to change what strategy you would use.”
In countries where rollout has been very slow compared to viral transmission, the models show that taking the direct approach and reducing mortality — by first vaccinating older people and others who are at higher risk — is the best way to move forward. But “if you’re South Korea, Taiwan, New Zealand” or somewhere else where transmission is under better control, Larremore said, “you have a totally different set of options” because the race against the virus looks so different. He and Bubar showed that similar shifts in strategy could rely on other factors, such as different reproduction numbers and overall transmission rates.
Now, though, the debate over vaccination concerns not just which people to vaccinate but also how and when to do it. The two approved vaccines in the U.S. each require two doses to deliver their full 95% efficacy. But given how slowly those vaccines are being distributed, researchers have begun modeling other scenarios, including giving as many people the first dose of the vaccine as possible, rather than setting aside half of the available doses to absolutely ensure that people who receive their first dose will receive their second on schedule.
That strategy has the advantage of ramping up baseline protection in a population: The first dose confers only about 52% protection, which isn’t satisfactory by the usual vaccine standards but could slow the spread of infections enough to prevent more cases and deaths in the long run. But it’s also gambling on the likelihood that enough second doses will be available when they are needed. People who receive their second dose later than intended may never get the full measure of vaccine immunity, and some researchers worry that widespread delays in producing full immunity could give the virus more opportunities to mutate and “escape” a vaccine’s control.
SEIR models have helped to quantify that gamble. Assuming certain dose efficacies, waning efficacy between doses over time, and other factors, the models have shown that trying to deploy as many first doses as possible can avert around 25% more COVID-19 cases (and sometimes more) over eight weeks than setting aside half of available doses can. In fact, the researchers found that only in the worst-case scenario — if the first dose of the vaccine had a very low efficacy and if the vaccine supply chain collapsed — would setting aside half the doses for the future be the better alternative.
Ferrari points out that this trade-off is nothing new: He’s seen it in his own work on measles and meningitis outbreaks, and in colleagues’ work on cholera, yellow fever, polio and other diseases. The mathematical models are straightforward, he says, and they show that in the midst of an outbreak, the emphasis should always be on quickly vaccinating as many people as possible, even if it means sacrificing some of the efficacy of the vaccination campaign.
Such models have been instrumental in leading the U.K. — and now the U.S. — to adopt that plan. Perhaps they wouldn’t have if the virus hadn’t “just put on rocket boots,” as Larremore put it, or if the vaccine rollouts had happened more efficiently in the first place. But that’s why models have to take into account so many possibilities and uncertainties. (There are other open questions, too, like how long immunity will last, and whether COVID-19 will be a one-time crisis or a seasonal ailment like the flu, which will affect future decisions about how many vaccines to continue buying and how to prioritize them.)
“The math is simple,” Ferrari said. “Where the math meets the real world is where the complications come in.”
Going forward, there are still questions about human behavior to reckon with. Because of disabilities, poverty or other obstacles, some people may not be able to get to a vaccine distribution center, or they may be hesitant about getting a vaccine at all. As vaccines protect those most susceptible to COVID-19, “we’ll see mortality drop — along with the coming of spring and the opening of more of the outdoors to people,” Larremore said. “I think we’re going to see a lot of pressure put on officials to really reopen things.” People will start to act differently regardless. Will they get less careful or take more risks after getting a first dose or a second, or after seeing more and more people receiving vaccines? How will that affect transmission and subsequent vaccination and intervention strategies?
As with so many decisions that have characterized the COVID-19 pandemic, it’s still true that, while one strategy might have a bigger impact for all of society, it might not be beneficial to certain individuals — say, those who could get a significantly delayed second dose. It places at odds “the perspectives of the medical doctor who sees the patient in front of her,” Larremore said, “and the public health modeler who sees how things are shifting at a very broad scale.”
That’s something that all modelers have encountered. “I can type in these numbers, or see the results saying this number of people might die if [these are] the groups that we choose to vaccinate,” Bubar said. “Just looking at it as a coding simulation, it feels very impersonal. But then we turn on the news and we see the number of actual people that have died every day, and it’s obviously very personal and very shocking.”
That’s why she and Larremore have also tried to incorporate questions about fairness and ethics into their vaccine prioritization models, including a strategy of combining vaccine rollout measures with antibody test results, particularly in areas hit hardest by the virus.
Meanwhile, the pandemic has stolen attention from other health issues, for instance through disruptions of major health care services — including, in many countries, vaccination programs for other diseases. Ferrari has been analyzing how these disruptions will affect measles outbreaks around the world over the next couple of years. Some of his work has already helped to prompt Ethiopia to move ahead with planned measles vaccination programs and other health care services; he’s currently doing more modeling work to determine when and how other regions should resume those practices.
Looking Forward, Looking Back
Researchers expect to be dissecting what happened during the COVID-19 pandemic for years to come. They will comb through the massive numbers of models that were generated and try to account for what worked, what didn’t and why. But there are key lessons they are already taking away from the experience in preparation for the inevitable next pandemic.
One is that they should take advantage of new, potentially rich streams of data from cell phones and other sources, which can provide detailed information about people’s real behaviors. Another is that certain kinds of problems are most easily conquered by dividing them up among teams, sometimes spanning several disciplines.
To make that possible, Manrubia and other researchers have called for national and worldwide programs dedicated to epidemiological forecasting and pandemic science. “We need to undertake a global program, similar to those meteorological institutes that you have in all countries,” Manrubia said. “This is something that doesn’t exist at the epidemiological level. And we need that, at the world scale.”
Such a program might guide the development of extensive systems for data collection and sharing, as well as infrastructure for rapid testing, contact tracing and vaccine production. It could frame more coherent strategies for when and how to use certain types of models for optimal results. It could also establish networks for helping experts in diverse fields to connect, and it could offer protocols for integrating their areas of expertise into informed decision-making processes. The COVID-19 pandemic has broken ground for building those capabilities.
But the other crucial lesson of COVID-19 has been that epidemiologists need to communicate the proper uses and limitations of their models more effectively to decision-makers and the public — along with an appreciation of what the uncertainties in those models mean. The frustrating challenge is that researchers are often already offering these explanations, but the public and its representatives tend to want more certainty than science can provide. And when governments decide to disregard researchers’ best counsel and clutch instead at specious but popular policies, it isn’t clear what scientists can do about it.
Drake says he had hoped that U.S. policy leaders understood how measures like lockdowns could create time to formulate a national response to the pandemic. “I thought we did the right thing by locking down. And then we squandered [it]. We bought ourselves a month,” he said. “But we didn’t do anything with that time.”
Jewell is also outraged at what he called the “shambolic” U.S. response. “There really should be a national strategy: If you’re in an area with this level of community transmission, this is what your schools could do,” he said. Instead, “there is no plan. There is no strategy. Everyone — every campus and every school system — is on their own.”
He points accusingly at “the shocking performance of the CDC.” He doesn’t blame individual researchers there, but “somehow, politically, the CDC has been completely compromised,” he said. “We always used to turn to the CDC: ‘Give us advice! What do we do?’ They were getting the best quality data.” But during this pandemic, that hasn’t happened. “They’ve given terrible advice.”
Drake recognizes that “it’s a policy decision, not a scientific one, as to what tradeoff you’re willing to accept in terms of the cost to the country and the number of lives lost — how many deaths could have been averted, what are we willing to pay for those.”
“But from my vantage point,” he continued, “much of the death and illness that we’ve seen in fact could have been prevented.” The models can warn us about fatalities to come, but we have to be willing to learn how to listen to them.