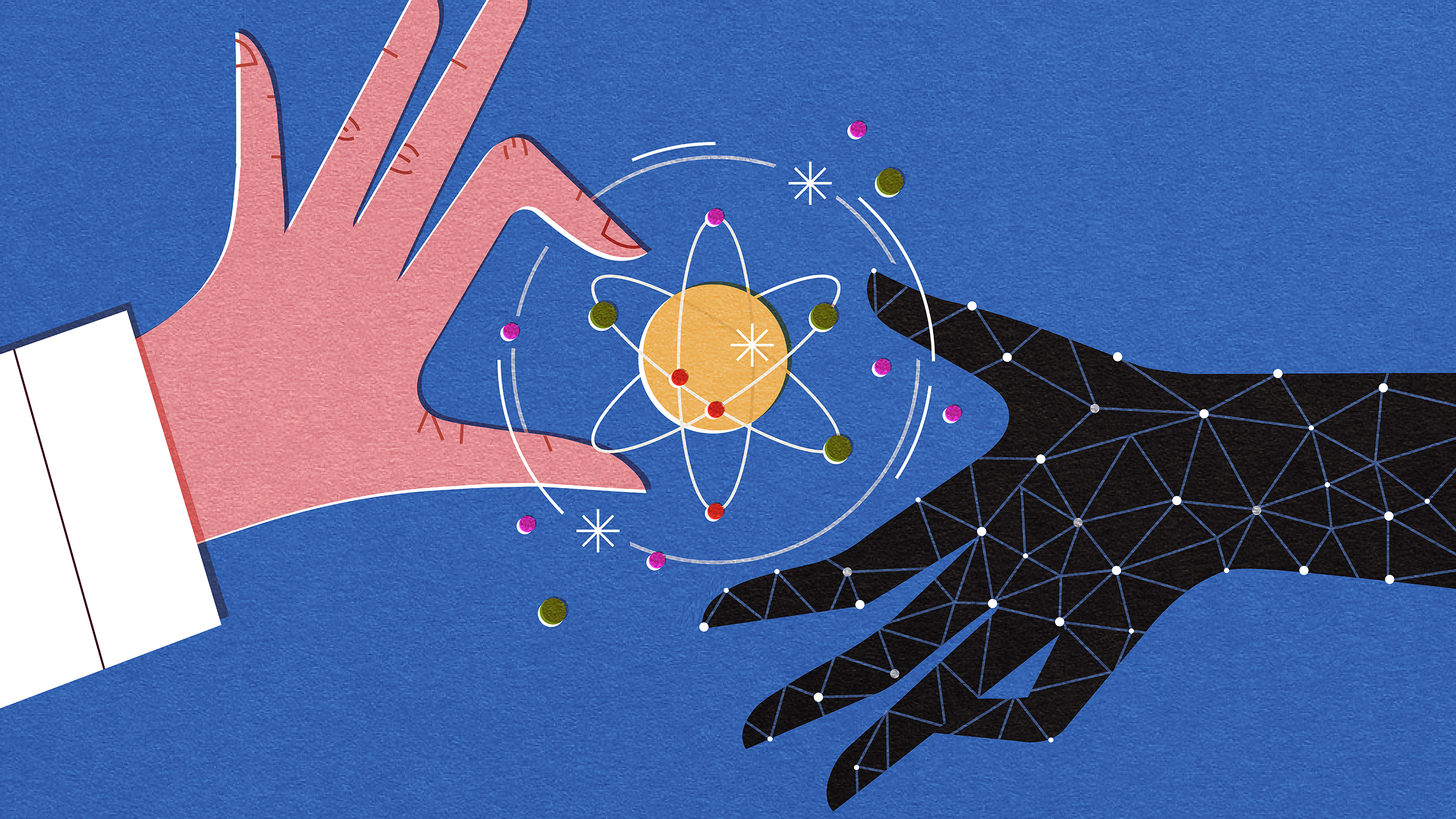
Sally Caulwell for Quanta Magazine
When Mario Krenn was studying quantum physics at the University of Vienna, he was trained in a particular way of designing new experiments: “You go to a blackboard, and you think very hard,” he said. In 2014, Krenn was trying to come up with a way to observe a particular quantum state. A typical setup would involve sending a laser beam through a finely calibrated array of lenses, crystals and mirrors. But the specific arrangement is up to the physicists. “Usually, an answer emerges,” Krenn said. Human reasoning is reliably clever magic.
Except this time, it wasn’t. Krenn and his fellow doctoral students had spent months gathering around the blackboard, doodling various setups and debating the theory. No answer had emerged.
But late at night, Krenn had begun working on a different approach to brainstorming. A few months before, he had read a paper from IBM researchers about an artificial intelligence system that wrote recipes. It was meant as an exercise in what the authors called “computational creativity.” With recipes such as “Caymanian plantain dessert,” which paired papaya salad with lime cream and coconut panna cotta, it was meant to explore what people generally mean when they say something is “creative” — a term that is often defined in terms of novelty and value.
Krenn, like most people, had mostly thought of creativity as a form of magic — something that was untouchably abstract. But the IBM researchers had made a claim that creativity is quantifiable. Therefore, it could be manipulated, or even optimized, by a computer. The idea thrilled him. Krenn decided he wanted to create new recipes in physics — to turn basic lab ingredients into new experiments. He designed a program to do this for him, which he named Melvin.
One evening, Krenn plugged his stubborn quantum problem into Melvin and let it run overnight. The next morning, after the machine spat out a proposal, he emailed it to his adviser, Anton Zeilinger, who would later win a Nobel Prize for his work in quantum physics. The reply came immediately: He liked it.
The experimental design was counterintuitive, Zeilinger observed. It was asymmetric, while the quantum state they desired was the opposite. “His explanation for why we didn’t find it [ourselves] was that we were biased in some way,” Krenn said. Human reasoning had apparently gotten in the way.
Quantum science being finicky, it would be four more years before the experiment designed by Melvin bore fruit. (It worked.) From there, Krenn’s colleagues mostly went back to the blackboard. Robert Fickler, who worked with Krenn on the experiment and is now a professor of physics at Tampere University in Finland, had been pleased to get the experiment moving along, but he told me he believed Melvin’s advance to be more about speed than creative insight. “I thought this was nice, but I felt we could have come up with it as well,” he said. “But we didn’t.”
Krenn, on the other hand, decided that it was time to ditch the traditional lab entirely. “I prefer my programs,” he said. He first went to work in a materials research lab, where AI discovery tools are more regularly used than they are in quantum physics. Then in 2021, he founded what he called the Artificial Scientist Lab at the Max Planck Institute for the Science of Light. (Krenn will soon move it to the University of Tübingen.)
Over the years, he has worked to extend Melvin beyond quantum experiments and into other realms of physics, searching for insights and connections that people have missed. “There’s a space for all physics experiments, and at some random location there’s a fantastic new microscope or gravitational wave detector,” he told me. Combine that expert domain knowledge with a large, general-purpose language model such as ChatGPT, and you’re on the road, Krenn thought, to delivering what the name of his lab promises.
The dream chased by academics like Krenn, as well as tech giants and startups raising money on the prospect of “scientific superintelligence,” involves folding AI into the creative aspects of science. Krenn, for example, hopes to create a system that would combine expert scientific systems, such as his physics simulators, with large language models that could sift through all the world’s knowledge and come up with new ideas and how to test them. Perhaps robots could then follow through on the experiments.
Researchers have been using AI-adjacent techniques such as machine learning as a data-processing tool for many years, often with terrific results. In addition, scientists now regularly report using generative AI to sift through papers to conduct high-speed literature reviews, tackle annoying bits of code, or help write emails.
Now the proposition has changed. Programs like Melvin and its successors, as well as advanced reasoning models from the likes of Google, promise help not just with composing an email, but with developing new research leads to pursue. AI is morphing from a data analysis tool to a tool for creativity. In doing so, it’s integrating itself into the heart of the scientific process, with consequences that are as far-reaching as they are hard to predict. What happens when we ask machines not just for the best way to learn things, but for the best things to learn?
Misery and Efficiency
In 2022, Aidan Toner-Rodgers, a doctoral student in economics at the Massachusetts Institute of Technology, got a rare opportunity to study how AI could shape what it means to be a scientist. A company working in materials discovery began assigning a new generative AI tool to more than 1,000 of its researchers. The hope was that the tool would help the researchers come up with new materials to test. Fortunately for Toner-Rodgers, the rollout would happen in waves, so some scientists would have access to the tool while others would not. As a result, there were built-in test and control groups.
The results of the experiment were striking. In the AI-equipped group, productivity soared. Those scientists discovered 44% more materials, obtained 39% more patents, and created 17% more products than the control group. High-achieving scientists — those who had already patented the most materials — came up with new ideas even faster, with the top decile improving their output by 81%, likely because they had the intuition to toss aside the AI’s worst ideas and test only the good ones.
But the AI doled out misery along with efficiency. More than 80% of the scientists who used AI said their job satisfaction had gone down. Based on interviews with his subjects, Toner-Rodgers hypothesized that it was because the researchers had lost the most creative portion of their job. “I couldn’t help feeling that much of my education is now worthless,” one of the scientists told him. “This is not what I was trained to do.”
To Philip Romero, a protein engineer at Duke University, the experience of those industrial researchers recalled earlier debates about AI in his own field. When Romero was in grad school, people would scoff at AI tools, which they felt glossed over the most important task of structural biology, which was to understand a given protein’s function — whether it would bind to a particular antibody, say, or glow fluorescent green — by learning how it folded up.
Then one day, an AI — DeepMind’s AlphaFold — effectively solved the protein-folding problem. Perhaps some scientists felt their careers had been made obsolete, their purposes rewritten. But mostly, science — and scientists — simply moved on. Having a quick answer to how a protein folded did not, in fact, directly answer the deeper questions of how proteins actually function. It was simply a new tool in protein researchers’ arsenal, and a way for them to work much faster.
Now Romero could dream up a protein, armed with knowledge of how it would fold, and get a head start on the hard work of analyzing what that meant. “I think satisfaction is way up,” he said.
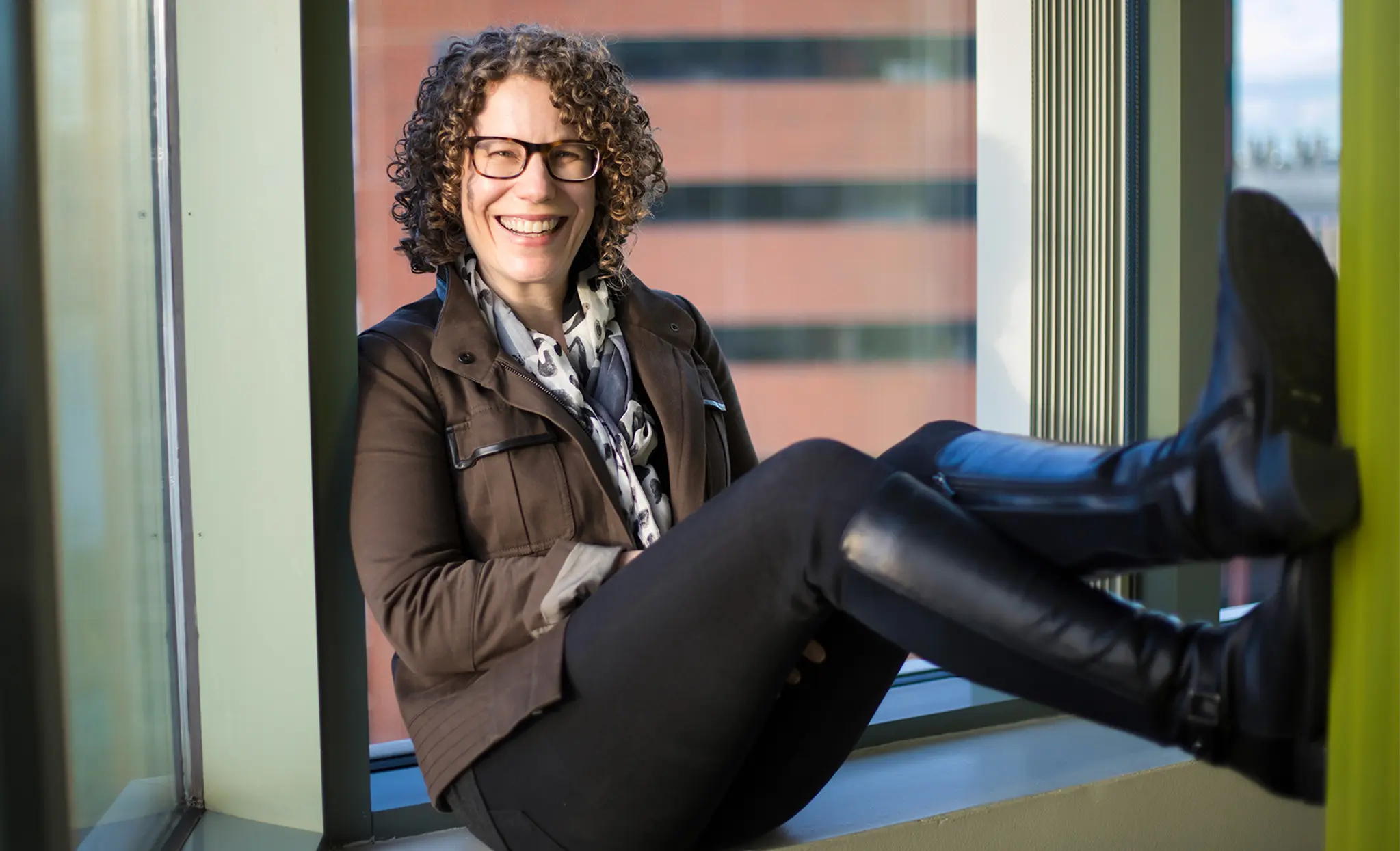
Jennifer Listgarten, a computer scientist who focuses on questions in biology, wants to be clear about what makes AI exceptional in certain limited cases.
Courtesy of Jennifer Listgarten
Perhaps that satisfaction has more to do with autonomy, suggests Jennifer Listgarten, a computer scientist at the University of California, Berkeley, who works closely with Romero and others to apply AI to biological questions. The industrial scientists wound up feeling more like technicians or lab assistants, carrying out the machine’s creative dreams rather than their own. “Everybody knows that the way to get your grad students to work on an idea is to have them think it’s their idea,” she said with a laugh. AI engaged in no such flattery.
Academics should, in theory, have more freedom to maneuver. Listgarten argues that they are a little like artists — free to experiment when new ideas and tools arrive, to start asking new questions and see “how it unleashes new forms of creativity,” she said. But force them to use it? If the experience of the corporate researchers tells us anything, it’s that doing more and doing it faster isn’t everything.
The AI Idea Machine
While tools such as AlphaFold have provided AI’s most visible scientific successes, to some the full promise of AI goes beyond mastery of narrow tasks. Researchers are imagining AI as a participant in the scientific process, providing suggestions for new research directions or validation of old ones — something between a tool and a colleague.
Such was the idea behind the “AI co-scientist,” which Google announced earlier this year in the hopes of producing novel hypotheses for scientific mysteries, along with ways to test them. The platform features AI agents that come up with potential answers to a specific research question. These agents then debate their theories and rank how strong they consider each to be, refining the ideas in the process.
Prior to the announcement, Google had asked José Penadés and Tiago Dias da Costa, two microbiologists with Imperial College London’s Fleming Initiative, to test the platform out. The pair decided to ask the co-scientist a question they had already answered in their labs but not yet published, about a way that a type of virus spreads its genetic information around. They sent their question to the co-scientist and were shocked when, amid a collection of not very good ideas, it gave them the very same hypothesis they had recently tested and found to be correct.
One reason for his surprise, Penadés told me, was that the reasoning skills of generative AI tools are still quite weak. This is why, despite their wide-ranging knowledge and fluency with language, they often struggle with basic math or working through hypothetical situations that don’t appear in their training data. It’s also why people have every right to be skeptical of any machine that purports to reason scientifically.
To Penadés, the AI had essentially picked up the puzzle pieces of different discoveries over the years, put them into place, and identified where the next piece should go. What was impressive, to him, was that it could make the next logical connection by rummaging through the scientific literature and finding a relevant insight. This was a bit like finding the final puzzle piece hiding in the couch cushions. It had taken Penadés and Costa ages to come to the same answer. It was a case, Costa thought, where their own thinking had gotten “formatted,” or stuck in a rut. Not unlike Krenn failing to find the right quantum setup, Costa had long allowed his own intuition to get in the way of seeing an idea that was, in retrospect, fairly obvious. The AI was able to make a leap.
The researchers came away from the experience thinking that what Google had demonstrated was essentially a superpowered search engine. Its capabilities beyond that were unclear. “First of all, you need to ask a question, which is the most valuable thing,” Penadés said. “Can a machine ask a very good question? I don’t know. Can a machine interpret things in a way that forgets about what is published and open a new way of research? I don’t know.”
But in the meantime, he thought, there was a lot to gain from an AI that could find the missing puzzle pieces. “It’s a very smart collaborator,” Penadés said. The question, in their minds, was how much could be discovered through this form of machine creativity. In the aftermath of the testing period, they asked their doctoral students and postdocs to act as reviewers for the AI’s output, poring over the questions it generates. Somewhere in the flood of new ideas, there might be new hypotheses worth testing.
Lessons and Limits
Last year, Krenn devised his own experiment to test the quality of AI hypotheses: He would train an AI on millions of research papers across a wide variety of subjects and instruct it to generate personalized research ideas and potential collaborations. Then, he would ask his colleagues across the Planck Society to tell him how good the AI’s ideas really were.
Among those colleagues was Ana Bastos, an Earth systems scientist at Leipzig University. She sent him a curt reply. “Of the many problems that current science faces today, I do not see how accelerating output of research ideas powered by AI would solve them,” she wrote. “In fact, I think it would rather contribute to the trend of science becoming less disruptive, less human and less diverse.” She declined to participate.
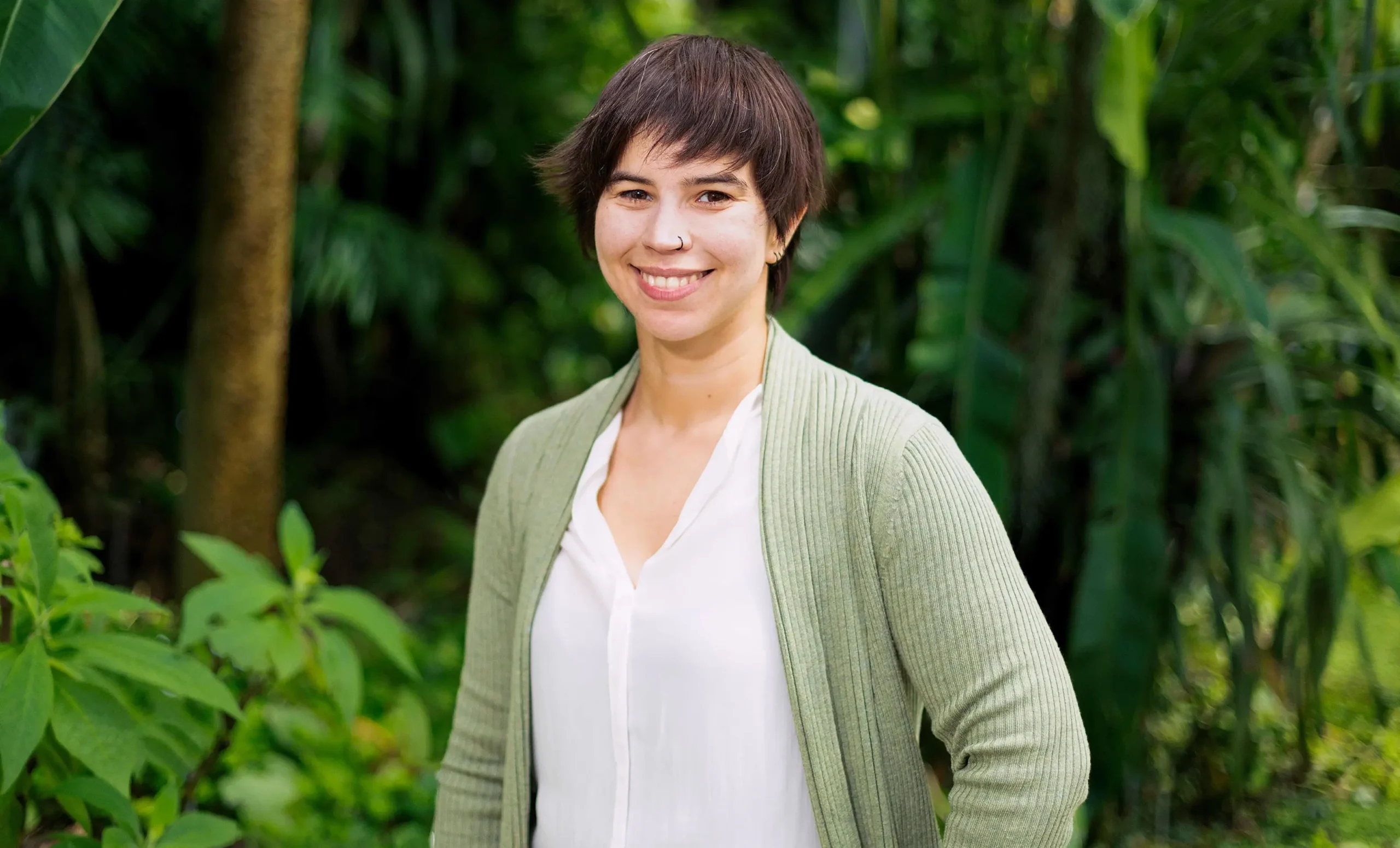
Ana Bastos worries about what widespread implementation of AI will mean for science.
Antje Gildemeister
To Bastos, the invitation was the latest of many unwelcome intrusions of AI into her life as an academic. She recalled a recent meeting where a colleague had commented on improved AI weather forecasts, which have recently begun to outperform traditional physics-based models for some tasks. The colleague predicted that they would solve climate modeling in five years using AI, and that soon they could “throw physics in the trash bin.”
That idea stunned Bastos, who regularly uses machine learning in her research. Even great AI weather-prediction models will tend to downplay the most extreme events, she said. They also may struggle as climate patterns change and underlying assumptions about the atmosphere — implicit in the data used to create those models — become obsolete. Not only that, the AI-based forecasts work best for near-term weather models that have great data sets to build from. Long-term climate modeling relies on data that is much scarcer and more uncertain.
Her colleague’s unchecked optimism — a kind of fatalism about the power of AI — made Bastos wonder if academics are adopting AI with as much autonomy as they might believe. The tool was being forced on the artists. She had noticed that grants and journal publications come more easily for work that uses AI. She wondered: What research isn’t getting funded as a consequence? What isn’t getting published?
She has seen a subtle creep in the way people rely on AI-powered literature reviews without checking the individual citations. In the future, what might prevent people from using AI to rate papers for publication, or to decide who gets funding? “I just think this acceleration of the curve is going to make everything worse,” Bastos told me.
To Listgarten, the Berkeley computer scientist, efficiency is one of the great promises of AI. It can accelerate science in a way that otherwise wouldn’t be possible. She compares it to the internet or the microscope. “The whole point is to produce results,” she said.
But an overlapping set of concerns drove her into a frenzy of writing one Sunday morning. She had been getting lots of inquiries about how AlphaFold and ChatGPT would “solve” science across a wide variety of domains. By that afternoon, she had completed a draft that would become an article in Nature Biotechnology called “The Perpetual Motion Machine of AI-Generated Data and the Distraction of ChatGPT as a ‘Scientist.’” It was about as close as a Nature journal gets to publishing a polemic.
She observed that many people, including scientific leaders, fail to realize what makes AI exceptional in certain limited cases such as AlphaFold. That project required an enormous set of meticulously labeled data. There, the AI was able to piggyback on decades of published experimental studies on protein structure that had cost about $20 billion to produce.
Not every field has the data that protein folding does or involves questions that are so well-suited to AI training. In the absence of either, people are looking for shortcuts, such as synthetic data created by AI to train other AI systems. Or they hope that large language models will demonstrate robust understanding, or even produce novel insights, in domains in which they were not by any means masters.
That approach, Listgarten argued, is bound to disappoint. In the search for the all-purpose machine, the oracle to understand the things that people cannot yet comprehend, there’s no getting around the need for data. “We’ll just need to get back to the bench and do more experiments,” she said.
The Next Leap
A decade after Krenn developed Melvin, the grad students who worked on the project meet on Zoom every few weeks to catch up and gossip about quantum physics.
Fickler was one of the more than 100 guinea pigs for Krenn’s survey of AI-generated research ideas. When the survey results arrived, most of the ideas were given a 1 on a scale of 1 to 5. But about a quarter were rated a 4 or 5 — that is, “interesting” or “very interesting.” To Krenn, 25% is a pretty good starting point. “I’m not sure I can produce so many interesting ideas,” he said.
It was all very much an experiment, and just because an idea was rated well, it didn’t mean anyone had to pursue it — that’s the beauty of science, after all, the choice of inquiry. They were just more ideas to throw in the pile. But it was a sign, Krenn said, that there was much more creative insight to mine along this current path of making surprising connections across science.
Could such a connection turn into a strikingly creative idea, one that simultaneously makes sense of all experimental evidence while challenging what we know? That’s unclear, even to Krenn. “It’s very difficult to see how such programs can come up with paradigm shifts” such as what Albert Einstein did with his profoundly creative theory of gravity, he said. But then again, he thought, we don’t fully understand how humans come up with genius insights, either. If creativity is ultimately more mechanical than magical, then a scientific creativity machine is not out of the question. “I’m very optimistic we can come up with it,” he said.
It’s an idea that still both impresses and worries Fickler, who often uses their video calls to prod his friend’s work with critiques and questions. “Maybe I don’t want AI to be that powerful,” he told me. “But very often Mario proves me wrong.”
For now, he is in the same boat as most scientists: trying to figure out how it all works. Fickler uses ChatGPT for occasional help with coding and for cleaning up grant proposals, since English is not his first language. It was a reluctant embrace; there was pressure, he thought, when everyone else had that edge, to move a little bit faster and sound a little bit better. What AI produces “sounds too good,” he said. “I like the mistakes; I like personality.” But it’s a hard thing to put away, he had found, once you start using it.