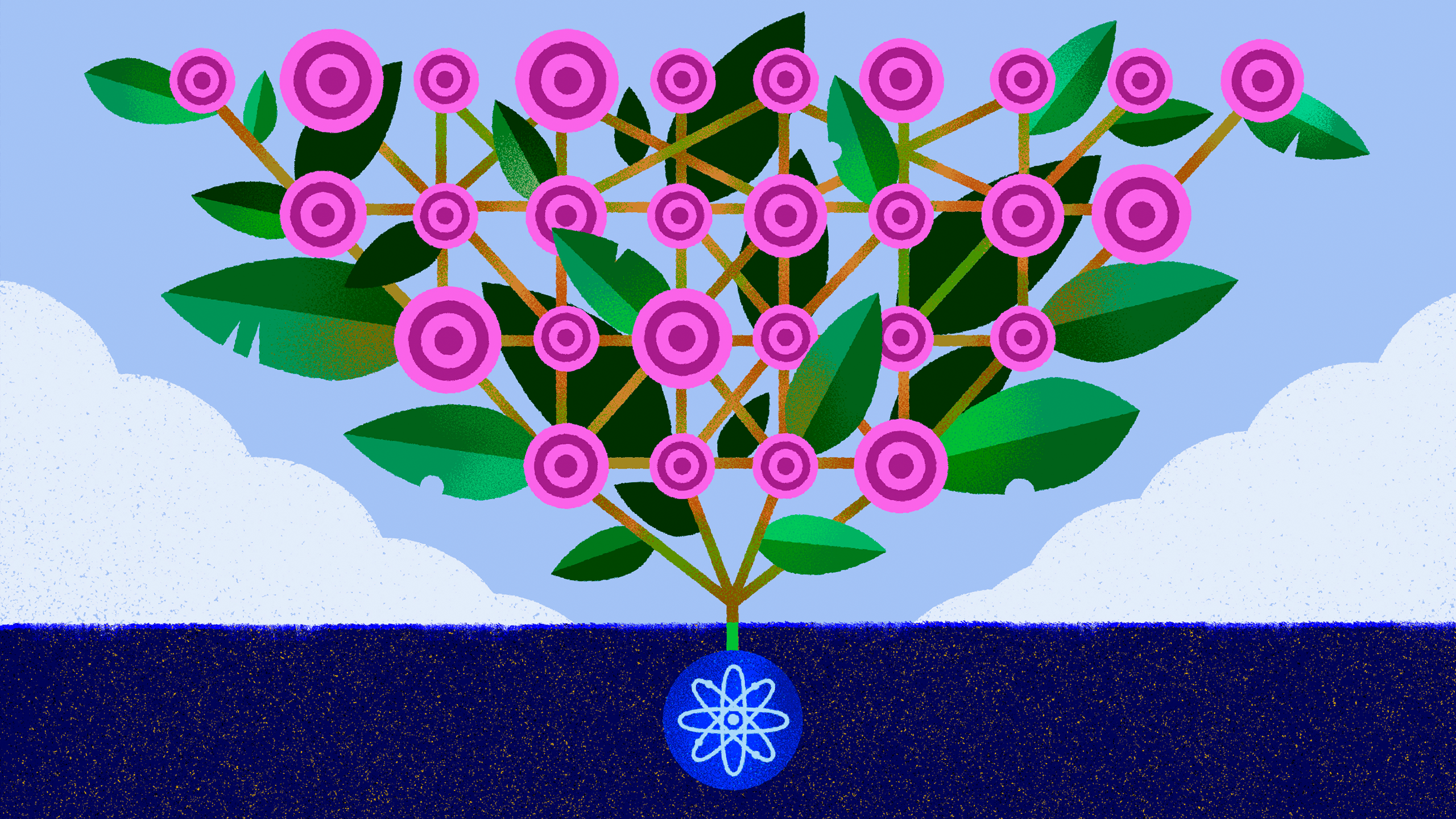
Irene Pérez for Quanta Magazine
Spin glasses might turn out to be the most useful useless things ever discovered.
These materials — which are typically made of metal, not glass — exhibit puzzling behaviors that captivated a small community of physicists in the mid-20th century. Spin glasses themselves turned out to have no imaginable material application, but the theories devised to explain their strangeness would ultimately spark today’s revolution in artificial intelligence.
In 1982, a condensed matter physicist named John Hopfield borrowed the physics of spin glasses to construct simple networks that could learn and recall memories. In doing so, he reinvigorated the study of neural networks — tangled nets of digital neurons that had been largely abandoned by artificial intelligence researchers — and brought physics into a new domain: the study of minds, both biological and mechanical.
Hopfield reimagined memory as a classic problem from statistical mechanics, the physics of collectives: Given some ensemble of parts, how will the whole evolve? For any simple physical system, including a spin glass, the answer comes from thermodynamics: “toward lower energy.” Hopfield found a way to exploit that simple property of collectives to store and recall data using networks of digital neurons. In essence, he found a way to place memories at the bottoms of energetic slopes. To recall a memory, a Hopfield network, as such neural nets came to be known, doesn’t have to look anything up. It simply has to roll downhill.
The Hopfield network was a “conceptual breakthrough,” said Marc Mézard, a theoretical physicist at Bocconi University in Milan. By borrowing from the physics of spin glasses, later researchers working on AI could “use all these tools that have been developed for the physics of these old systems.”
In 2024, Hopfield and his fellow AI pioneer Geoffrey Hinton received the Nobel Prize in Physics for their work on the statistical physics of neural networks. The prize came as a surprise to many; there was grumbling that it appeared to be a win for research in AI, not physics. But the physics of spin glasses didn’t stop being physics when it helped model memory and build thinking machines. And today, some researchers believe that the same physics Hopfield used to make machines that could remember could be used to help them imagine, and to design neural networks that we can actually understand.
Emergent Memory
Hopfield started his career in the 1960s working out the physics of semiconductors. But by the end of the decade, “I had run out of problems in condensed matter physics to which my particular talents seemed useful,” he wrote in a 2018 essay. So he went looking for something new. After a foray into biochemistry that produced a theory of how organisms “proofread” biochemical reactions, Hopfield settled on neuroscience.
“I was looking for a PROBLEM, not a problem,” he recalled in his essay, emphasizing the need to identify something truly important. “How mind emerges from brain is to me the deepest question posed by our humanity. Definitely a PROBLEM.”
Associative memory, Hopfield realized, was a part of that problem that his tool kit from condensed matter physics could solve.
In a normal computer, data is stored statically and accessed with an address. The address doesn’t have anything to do with the information that’s stored. It’s just an access code. So if you get the address even a little bit wrong, you’ll access the wrong data.
That’s not how humans seem to remember things. We often remember by association. Some cue or scrap of memory brings the full thing flooding back. It’s what happens when you smell lilacs and recall a childhood episode in your grandpa’s garden, or when you hear the first few lines of a song and find yourself belting out every word to a ballad you didn’t know you knew.
Hopfield spent years on understanding associative memory and translating it to a neural network. He tinkered with randomly wired neural networks and other potential models of memory. It wasn’t looking good until, eventually, Hopfield identified an unlikely key to the “PROBLEM.’’
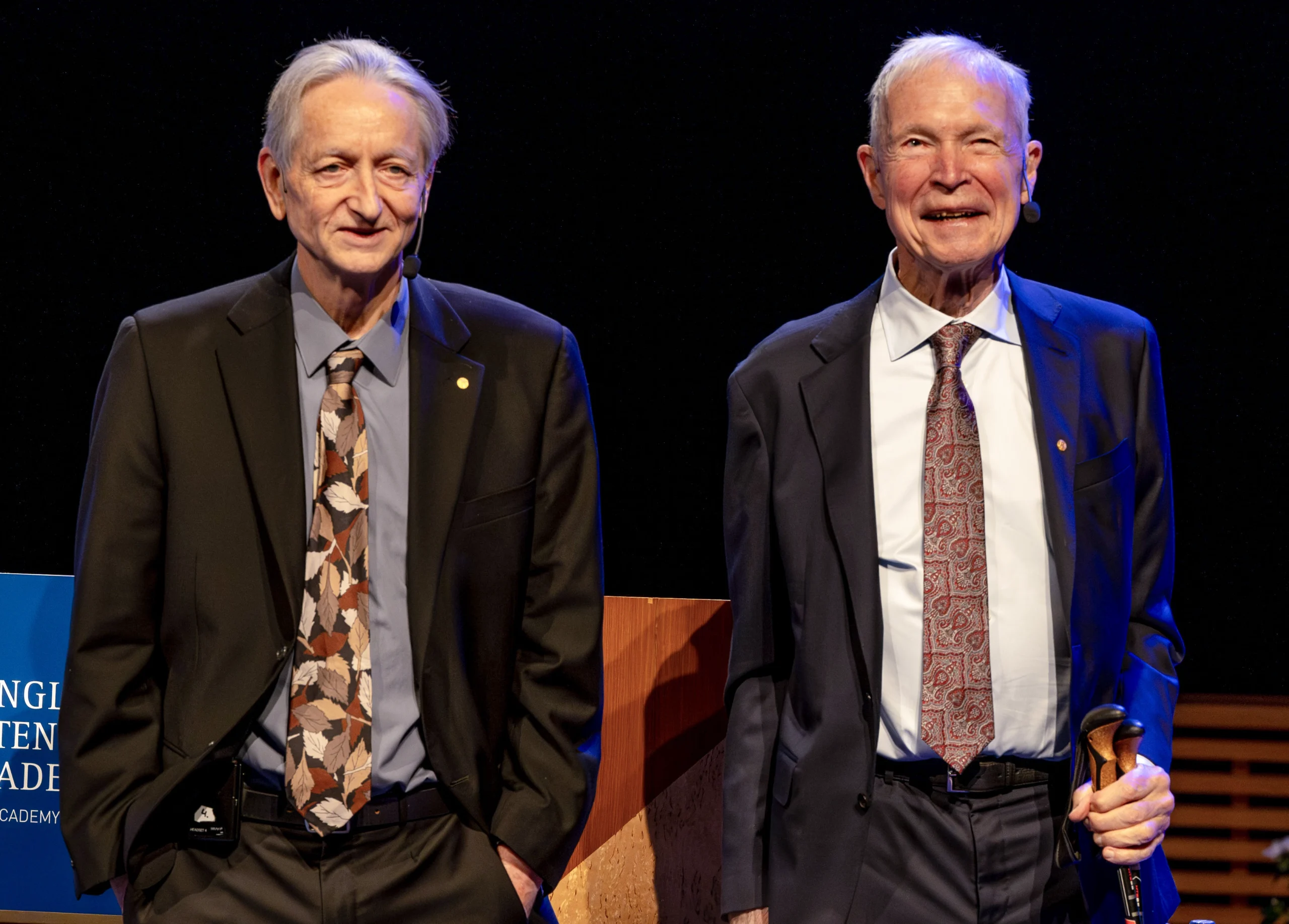
Geoffrey Hinton (left) and John Hopfield accepted the 2024 Nobel Prize in Physics at a ceremony in Stockholm in December. The prize honored their pioneering work on the earliest neural network models, which were based on the physics of spin glasses.
Wikimedia Commons
Spin Glasses
In the 1950s, scientists studying certain dilute alloys such as iron in gold realized that their samples were doing some strange things. Above a certain temperature, these alloys behave similarly to a normal material such as aluminum. They aren’t magnetic on their own, but they do interact weakly with external magnetic fields. For instance, you can use a very strong magnet to move an aluminum can, but aluminum itself can’t work as a magnet. Usually, materials such as aluminum lose their magnetization as soon as the external magnet disappears. But below a certain temperature, spin glasses do something different. Their transient magnetization sticks around, albeit at a lower value. (This isn’t the only weird thing that spin glasses do; their thermal properties are also puzzling.)
Around 1970, condensed matter physicists started to get a theoretical handle on these materials by tweaking physicists’ go-to model of collective magnetic behavior: the Ising model.
An Ising model looks like a simple grid of arrows, each of which can point up or down. Every arrow represents the intrinsic magnetic moment, or “spin,” of an atom. This is a simplification of a real atomic system, but by tweaking the rules by which nearby spins affect one another, the model can generate surprisingly complex behaviors.
In general, nearby arrows that point in the same direction have low energy, while arrows that point in opposite directions have high energy. If the spins are free to flip, the Ising model’s state will thus evolve towards a lower-energy state of alignment, like a ball rolling downhill. Magnetic materials such as iron end up settling into simple states with their spins aligned in either the all-up or all-down state.
In 1975, the physicists David Sherrington and Scott Kirkpatrick devised a model that could capture the more complicated behavior of spin glasses by modifying the rules of how spins interact. They randomly varied the interaction strengths between spin pairs and allowed each spin to interact with every other spin — not just its nearest neighbors. That change led to a rugged “landscape” of possible energy states. There were peaks and valleys corresponding to higher and lower energy configurations; depending on where the spin glass started off in this landscape, it would end up in a unique valley, or low-energy equilibrium state. That’s quite different from ferromagnets such as iron, which “freeze” into one of two orderly states with all spins aligned, and nonmagnets, whose spins fluctuate randomly and don’t settle down at all. In a spin glass, randomness gets frozen.
The Ising model is very much a toy model. Using it to try to predict anything about real materials is a bit like using a stick figure to plan a surgery. But remarkably, it often works. The Ising model is now a workhorse of statistical mechanics. Variations on its theme can be heard in just about every corner of the study of complex, collective phenomena — including, because of Hopfield, memory.
Spin Memory
A simple view of interacting neurons has a lot in common with an Ising model of magnetic spins. For one thing, neurons are often modeled as basically binary on-off switches; they either fire or they don’t. Spins, likewise, can point either up or down. In addition, a firing neuron can either encourage or discourage the firing of its neighbor. These variable interaction strengths between neurons recall the changeable interaction strengths between spins in a spin glass. “Mathematically, one can replace what were the spins or atoms,” said Lenka Zdeborová, a physicist and computer scientist at the Swiss Federal Institute of Technology Lausanne. “Other systems can be described using the same toolbox.”
To make his network, Hopfield started with a web of artificial neurons that can be either “on” (firing) or “off” (resting). Each neuron influences every other neuron’s state, and these interactions can be adjusted. The network’s state at any given time is defined by which neurons are firing and which are at rest. You can code these two states in binary: A firing neuron is labeled with a 1 and a resting neuron with a 0. Write out the state of the entire network at any given moment, and you’ve got a string of bits. The network doesn’t “store” information, exactly. It is information.
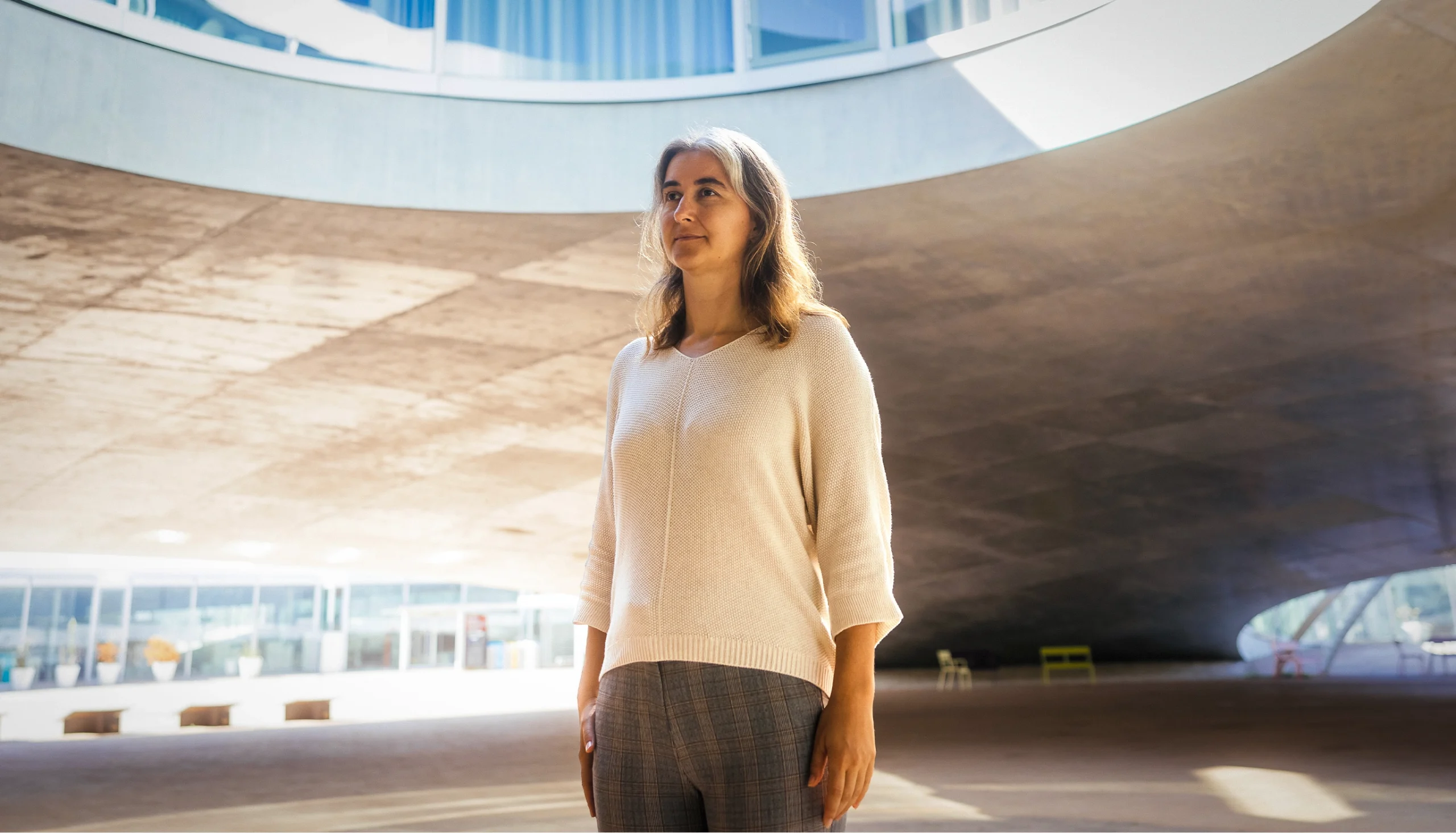
Lenka Zdeborová, a physicist and computer scientist at the Swiss Federal Institute of Technology Lausanne, studies how the physics of matter can help model the behavior of machine learning algorithms.
Samuel Rubio for Quanta Magazine
To “teach” the network a pattern, Hopfield sculpted its energy landscape by modifying the strengths of interactions between neurons so that the desired pattern fell at a low-energy steady state. In such a state, the network stops evolving and stabilizes in just one pattern. He found a rule for doing this inspired by neuroscience’s classic “neurons that fire together wire together” rule. He would tune up interactions between neurons that both fire (or both rest) in the desired final state and dial down interactions between mismatched pairs. Once a network is taught a pattern this way, it can reach the pattern again simply by navigating downhill through the network’s energy landscape; it will naturally reach the pattern when it settles into an equilibrium state.
“Hopfield made the connection and said, ‘Look, if we can adapt, tune the exchange couplings in a spin glass, maybe we can shape the equilibrium points so that they can become memories,’” Mézard said.
Hopfield networks can remember multiple memories, each in its own little energy valley. Which valley the network falls into depends on where it begins in its energy landscape. In a network that stores a picture of a cat and a picture of a spaceship, for instance, a starting state that’s vaguely cat-shaped will roll down into the cat valley more often than not. Likewise, starting the network in a state that recalls the geometric forms of a spaceship will usually prompt it to evolve toward the spaceship. That’s what makes Hopfield networks a model of associative memory: Given a corrupted or incomplete version of a memory, a Hopfield network dynamically reconstructs the whole thing.
Old Model, New Ideas
From 1983 to 1985, Hinton and his colleagues built on Hopfield’s work. They found ways to inject randomness into Hopfield networks to create a new type of neural network called a Boltzmann machine. Rather than remember, these networks learn the statistical patterns in training data and spin up new data to match those patterns — an early kind of generative AI. In the 2000s, Hinton was able to use a pared-down version of the Boltzmann machine to finally crack the stubborn problem of training “deep’’ neural networks consisting of multiple layers of neurons.
By 2012, the success of deep neural networks developed by Hinton and other pioneers was impossible to ignore. “It became clear that this is actually working amazingly well and just transforming the whole tech industry,” Zdeborová said. The generative AI models many of us now interact with every day, including large language models such as ChatGPT and image-generation models such as Midjourney, are all deep neural networks. They can trace their success back to curious physicists in the 1970s who refused to let the “useless” properties of spin glasses go unexplained.
Hopfield networks aren’t just part of AI’s past, however. Thanks to new ideas, these old models could be making a comeback.
In 2016, Hopfield and Dmitry Krotov of IBM Research realized that Hopfield networks weren’t just one model, but a whole family of models with different memory storage capacities. Then, in 2020, another team showed that a key part of the transformer architecture, the blueprint of most modern successful AI models, was a member of that extended Hopfield network family.
Armed with that insight, Krotov and his colleagues recently developed a new deep learning architecture called the energy transformer. Typical AI architectures are usually found by trial and error. But Krotov thinks energy transformers could be designed more intentionally with a specific energy landscape in mind, like a more complex take on a Hopfield network.
Though Hopfield networks were originally designed to remember, researchers are now exploring how they can be used to create. Image generators such as Midjourney are powered by “diffusion models,” which are themselves inspired by the physics of diffusion. To train them, researchers add noise to the training data — say, pictures of cats — and then teach the model to remove the noise. That’s a lot like what a Hopfield network does, except instead of always landing on the same cat picture, a diffusion model removes “non-cat” noise from a noisy, random starting state to produce a new cat.
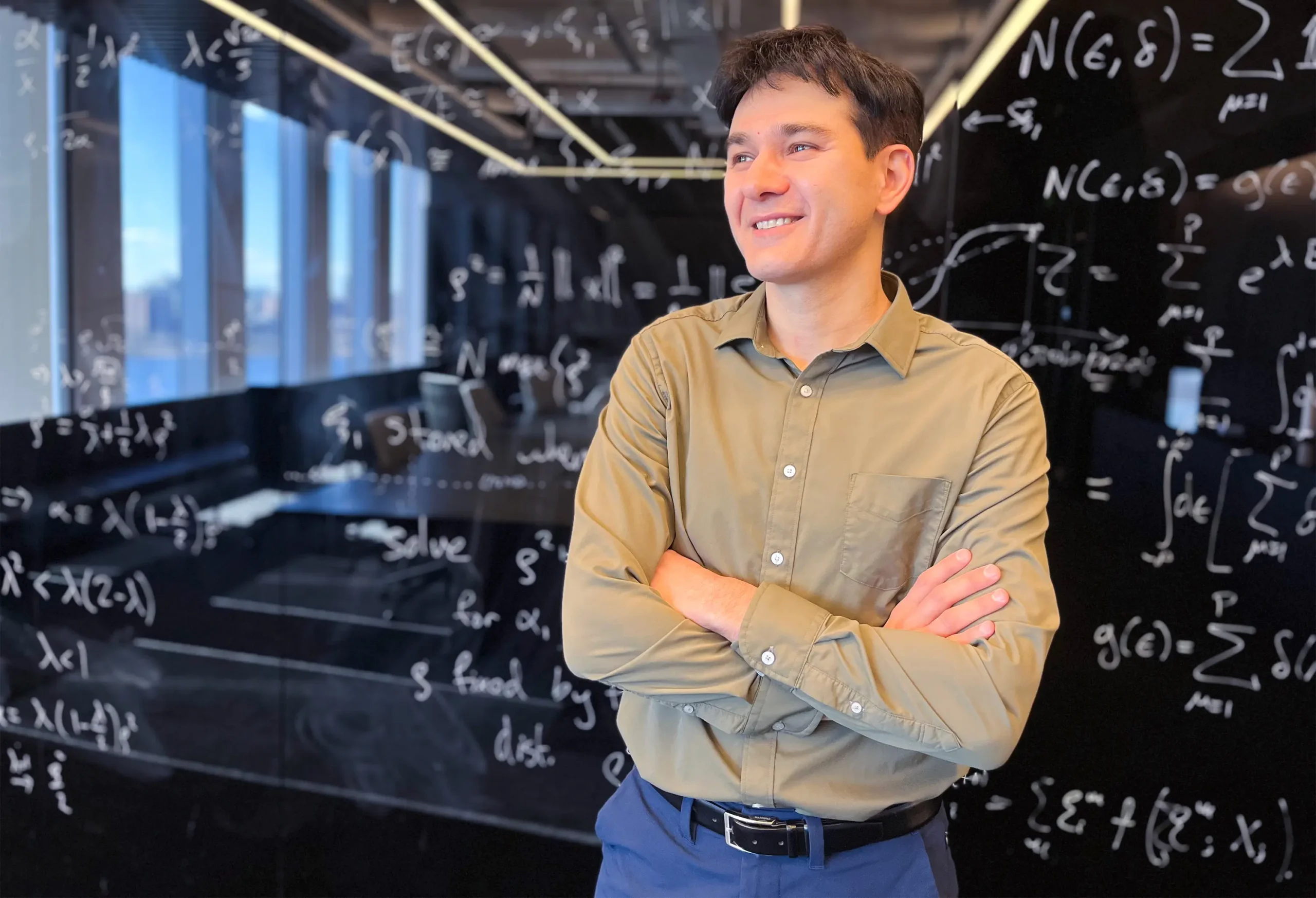
Dmitry Krotov, a computer scientist at IBM Research, has shown that some of the most advanced AI models in use today follow the same basic principle that Hopfield networks employed from the start.
Kim Martineau
It turns out that diffusion models can be understood as a particular kind of modern Hopfield network, according to Krotov and his colleagues, including Benjamin Hoover, Yuchen Liang and Bao Pham. And that approach can be used to predict aspects of these networks’ behavior. Their work suggests that feeding a modern Hopfield network more and more data doesn’t just saturate its memory. Instead, the model’s energy landscape gets so rugged that it is more likely to settle on a made-up memory than a real one. It becomes a diffusion model.
That a simple change in quantity — in this case, the amount of training data — can trigger an unexpected change in quality isn’t anything new for physicists. As the condensed matter physicist Philip Anderson wrote back in 1972, “more is different.” In collective systems, simply scaling up networks of interactions between parts can add up to surprising new behaviors. “The fact that [a neural network] works is an emergent property,” Mézard said.
Emergence in a deep learning architecture — or a brain — is as captivating as it is puzzling; there’s no universal theory of emergence. Perhaps statistical physics, which provided the first tools for understanding collective behavior, will be the key not just to using but also to understanding the inscrutable machine intelligences changing our world.