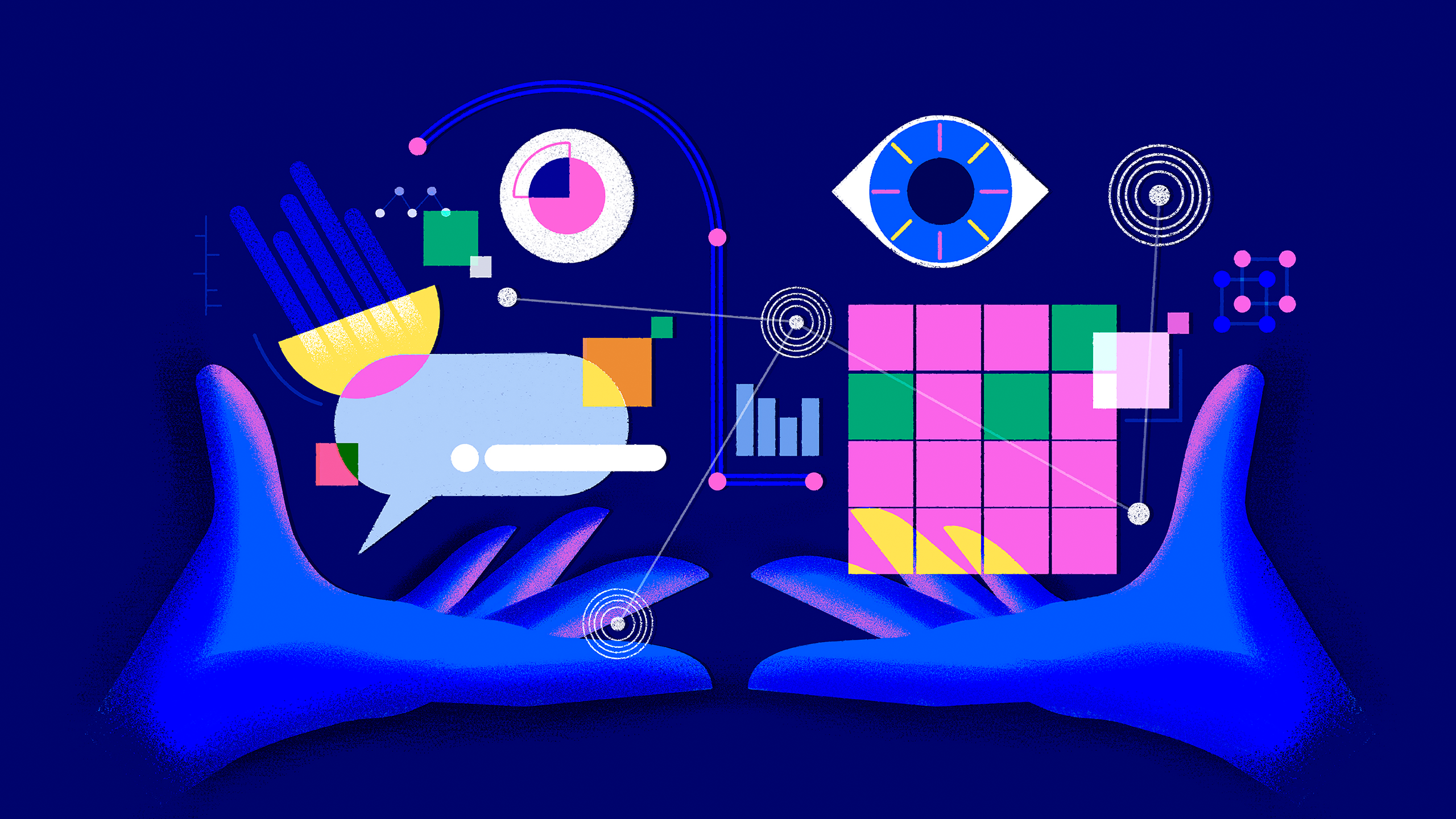
Irene Pérez for Quanta Magazine
Introduction
Artificial intelligence moves fast, so the first step in understanding it — and its role in science — is to know the lingo. From basic concepts like “neural networks” and “pretraining” to more contested terms like “hallucinations” and “reasoning,” here are 19 key ideas from the world of modern AI. Starting with …
* * *
Artificial Intelligence / “The science and engineering of making intelligent machines,” according to John McCarthy, who coined the phrase in 1955. Over the following decades, the ideas and practices associated with artificial intelligence have intersected with fields as diverse as cognitive science, machine learning, robotics and linguistics. The term AI now refers mainly to systems that use artificial neural networks. Specific AI applications — especially ones based on “generative” models such as AlphaFold3 or ChatGPT — are often simply called AIs.
Benchmark / A set of specific tasks designed to assess the performance of AI systems. They can also drive progress in research: In 2012, a benchmark called ImageNet helped establish the dominance of neural networks, and in 2018 GLUE did the same thing for transformer-based language models. They must be carefully designed to prevent skewed or misleading results. (For example, a language model called BERT appeared to ace a reasoning benchmark by exploiting the fact that the word “not” often appeared in correct answers; removing this quirk lowered BERT’s performance to no better than chance.)
Bias / Information in an AI system that influences its output in particular ways. Generative models trained on large internet datasets often contain negative biases: For example, in 2023, the AI image generator Stable Diffusion was more likely to depict “CEO[s]” as men with light skin. However, methods like reinforcement learning and symbolic regression can be used to give AI systems more productive biases, such as taking turns in a dialogue or respecting the laws of physics.
Embeddings / Long lists of numbers that neural networks use to represent particular relationships within their training data. They’re like coordinates: In a pretrained language model, the embedding for “dog” is geometrically similar to the embedding for “puppy,” and less similar to the one for “risotto.” But because these numerical values are assigned by a machine learning algorithm, they are rarely interpretable by humans.
Foundation Model / A phrase coined by Stanford University researchers in 2021 to describe AI models whose extensive pretraining makes them adaptable to a range of tasks (rather than being custom-built for specific applications, as is typical in machine learning). Image and text generators such as DALL-E and GPT-3 were among the first foundation models, but researchers are actively developing new ones in domains like physics, biology and robotics.
Generalization / The ability of an AI system to respond well to new inputs that were not part of its original training data. For example, a computer vision model trained to identify cats in images may perform well when cats face the camera, but fail to generalize when cats are shown in profile or upside down. Foundation models may be pretrained on vast data sets — including ones from scientific disciplines — to improve their generalization abilities, or bias them in useful ways.
Generative AI / Large neural networks, typically based on transformers, that can produce novel outputs such as writing or images. Generative techniques enabled the “AI boom” that followed the release of ChatGPT in 2022; they also power systems like AlphaFold, which predicts protein structures, and diffusion-based image generators.
Hallucinations / A common (but controversial) term of art for undesirable outputs produced by generative AI, such as when chatbots invent incorrect facts or when image generators create seven-fingered hands. No foolproof method for preventing hallucinations currently exists.
Inference / Shorthand for what happens when a trained neural network computes a prediction. Unlike humans, current models can only integrate new information during training, not during inference. This is why they have “knowledge cutoffs” that indicate when their training stopped (for example, October 2024 for Claude 3.7 Sonnet). However, increasing the amount of computation an AI system does at “inference time” (versus training) may improve its performance, especially on so-called reasoning tasks.
Machine Learning / A subfield of AI that uses algorithms to automatically “train,” or improve the performance of, other algorithms. In one popular approach, a mathematical function first measures how well an algorithm does at some predictive task (like classifying images) by comparing the algorithm’s predictions against a set of examples, called “training data.” The algorithm then gets tweaked to improve its predictions. This process is then repeated many times until the algorithm is able to make accurate-enough predictions when given new data. Algorithms trained in this way, such as neural networks, tend to perform better on inputs that are similar to their training data.
Matrix Multiplication / The basic arithmetic that powers modern AI — and a major source of its massive energy demands. Most of the computations happening within neural networks involve huge tables of numbers, known as matrices, being multiplied together countless times. Researchers have been trying to optimize this process for decades, including using AI to do so. Removing it altogether could radically improve AI’s energy efficiency.
Mechanistic Interpretability / An emerging subfield of AI research that focuses on understanding how neural networks process data by empirically probing, defining and verifying the internal mechanisms that produce their output. Experiments — sometimes inspired by neuroscience — may involve reverse engineering certain features that the network learned from its training data (for example, the phrase “Golden Gate Bridge”), computational circuits and individual neurons.
Model / A mathematical representation of a task, created by applying a machine learning algorithm to a training data set. For example, a large language model like GPT-3.5 (which powered the original version of ChatGPT) attempts to predict the next chunk of text in a given sequence — including sequences it wasn’t trained on. An AI model will automatically encode (or “embed”) any features in the data that happen to increase its task performance, regardless of whether those features are interpretable, correct or even relevant to the intent of the task itself.
Neural Network / The workhorse model of modern AI, which roughly mimics the connections in a mammalian brain by arranging mathematical functions called “neurons” into layers that process and pass on data to other layers. Each neuron includes “parameters,” numerical values set by a machine learning algorithm during training. The first layer of neurons encodes raw input, while hundreds or thousands of “hidden” layers — which may comprise billions or even trillions of parameters — perform calculations (typically matrix multiplication) that eventually produce a prediction in the output layer. [Check out our immersive explainer of how neural networks work.]
Pretraining / Training an AI model on a large set of diverse, often unlabeled data (like raw text scraped from the internet) to increase its ability to respond well to new inputs. A pretrained model is usually “fine-tuned” with additional training determined by its intended use. For example, to create ChatGPT, OpenAI pretrained a model on hundreds of billions of words collected from the internet, then used a technique called reinforcement learning with human feedback to optimize this model for generating chat-style dialogues.
Reasoning / A sequence of internal prompts (called a “chain-of-thought”) self-generated by some AI models at inference time, used to break down complex requests into simpler steps and (sometimes) produce more accurate responses. Because they consist of human-readable text, these “chains” can look remarkably like a bona fide reasoning process — but not everyone is convinced.
Reinforcement learning / A machine learning technique in which an AI system improves its performance by maximizing a “reward” signal through trial and error. Often used to train robots and game-playing agents, reinforcement learning is now helping models avoid hallucinations and enable behavior that resembles reasoning.
Synthetic Data / Data produced by generative AI that is then used as training data for other models. Researchers turn to synthetic data where there’s a problem with the quality or quantity of available training data. For example, a Stanford AI model can generate medically accurate chest X-ray images to correct for biases in existing data sets (such as a lack of female patients). However, over-training on synthetic data may cause AI models to “collapse” in a spiral of degraded performance.
Transformer / A type of neural network that breaks sequences of input into small chunks and learns contextual relationships between them so it can make predictions. These networks can be applied to a variety of tasks including document translation, text and video generation, speech recognition, game playing, computer vision and protein folding. Invented by Google in 2017, transformers became the basis for building the first large language models, and soon became the dominant architecture for contemporary AI systems.